The Role of Artificial Intelligence in eBike Maintenance
Artificial Intelligence (AI) is transforming the eBike industry by enabling predictive maintenance strategies that minimize downtime and reduce maintenance costs. Predictive maintenance for eBikes using AI involves using machine learning algorithms to analyze data from eBike sensors and predict potential failures before they occur. This proactive approach to maintenance offers numerous benefits, including increased efficiency, safety, and longevity of eBike components.
Traditional maintenance approaches, such as reactive and preventive maintenance, can be time-consuming and costly. Reactive maintenance involves fixing components only after they fail, which can result in extended downtime and safety risks. Preventive maintenance, on the other hand, involves regularly scheduled maintenance tasks, regardless of whether components need to be replaced or not. Predictive maintenance using AI, however, offers a more efficient and cost-effective solution.
By analyzing data from eBike sensors, such as battery health, motor temperature, and pedal assist levels, AI algorithms can identify patterns and anomalies in the data. These algorithms can then predict potential failures and provide recommendations for maintenance actions. For example, if an eBike’s battery is showing signs of degradation, the AI system may recommend replacing the battery before it fails completely, preventing downtime and ensuring the eBike remains safe to use.
Moreover, predictive maintenance using AI can lead to increased customer satisfaction and improved safety. By minimizing downtime and reducing maintenance costs, eBike manufacturers and service providers can offer a better customer experience. Additionally, predictive maintenance can help ensure the safety of eBike components, reducing the risk of accidents and injuries.
In summary, predictive maintenance for eBikes using AI is a game-changer for the eBike industry. By leveraging AI to analyze data from eBike sensors, manufacturers and service providers can predict potential failures before they occur, minimizing downtime, reducing maintenance costs, and improving safety. As the eBike industry continues to grow, predictive maintenance using AI will become increasingly important, enabling eBike manufacturers and service providers to offer a better customer experience and remain competitive in the market.
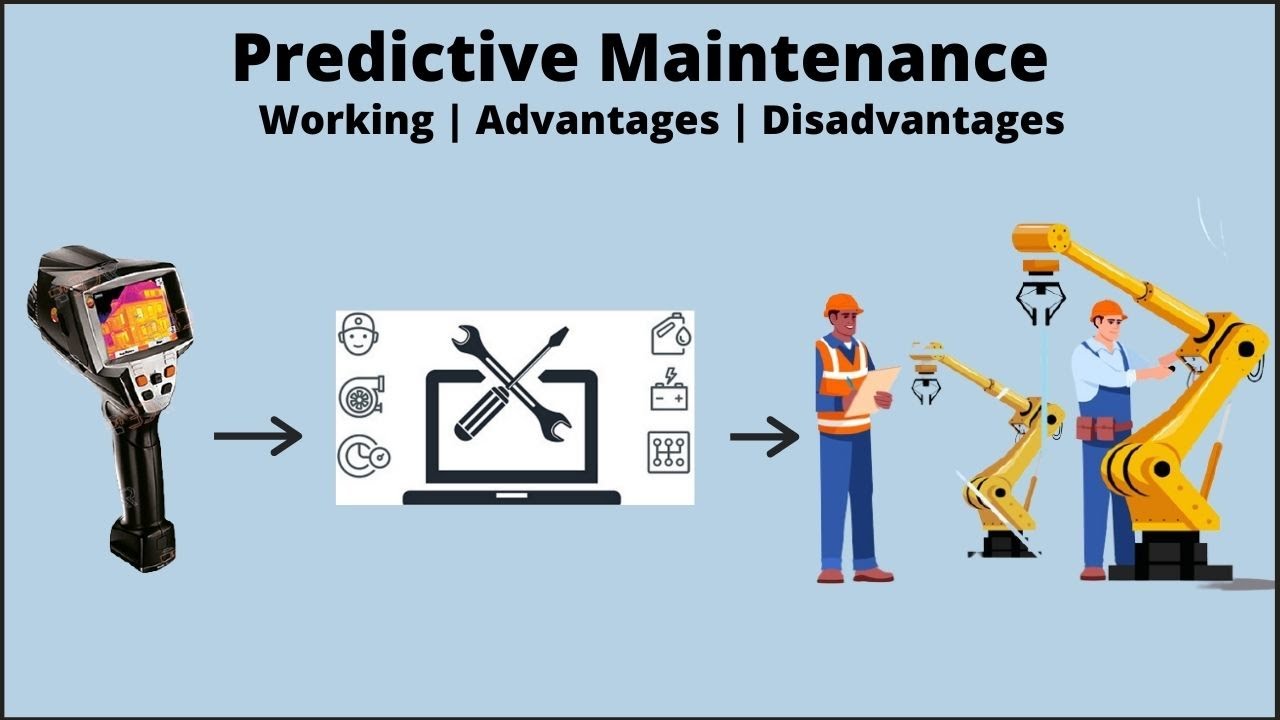
Understanding Predictive Maintenance for eBikes
Predictive maintenance for eBikes using AI is a proactive maintenance approach that leverages data and machine learning algorithms to predict potential failures before they occur. This approach differs from traditional maintenance methods, such as reactive and preventive maintenance. Reactive maintenance involves fixing components only after they fail, while preventive maintenance involves regularly scheduled maintenance tasks, regardless of whether components need to be replaced or not.
Predictive maintenance using AI, however, offers a more efficient and cost-effective solution. By analyzing data from eBike sensors, such as battery health, motor temperature, and pedal assist levels, AI algorithms can identify patterns and anomalies in the data. These algorithms can then predict potential failures and provide recommendations for maintenance actions. For example, if an eBike’s battery is showing signs of degradation, the AI system may recommend replacing the battery before it fails completely, preventing downtime and ensuring the eBike remains safe to use.
Predictive maintenance using AI offers numerous advantages over traditional maintenance approaches. For instance, it can help reduce maintenance costs by minimizing downtime and preventing unnecessary maintenance tasks. Additionally, predictive maintenance can improve safety by ensuring the timely replacement of components before they fail. Furthermore, predictive maintenance can increase efficiency by optimizing maintenance schedules and reducing the need for regular maintenance tasks.
Predictive maintenance for eBikes using AI is particularly important in the eBike industry, where components such as batteries and motors are subject to wear and tear. By leveraging AI to analyze data from eBike sensors, manufacturers and service providers can predict potential failures before they occur, minimizing downtime, reducing maintenance costs, and improving safety. As the eBike industry continues to grow, predictive maintenance using AI will become increasingly important, enabling eBike manufacturers and service providers to offer a better customer experience and remain competitive in the market.
In summary, predictive maintenance for eBikes using AI is a proactive maintenance approach that leverages data and machine learning algorithms to predict potential failures before they occur. This approach offers numerous advantages over traditional maintenance methods, including reduced maintenance costs, improved safety, and increased efficiency. As the eBike industry continues to grow, predictive maintenance using AI will become increasingly important, enabling eBike manufacturers and service providers to offer a better customer experience and remain competitive in the market.
How AI Enables Predictive Maintenance for eBikes
Predictive maintenance for eBikes using AI is made possible through the analysis of data from various eBike sensors. These sensors collect data on various aspects of eBike performance, including battery health, motor temperature, pedal assist levels, and more. By analyzing this data, AI algorithms can identify patterns and anomalies that may indicate potential failures before they occur.
AI algorithms can use various techniques to analyze eBike sensor data. For instance, machine learning algorithms can be used to identify patterns in the data that may indicate potential failures. These algorithms can be trained on historical data to identify patterns that are indicative of specific types of failures. Additionally, deep learning algorithms can be used to analyze large volumes of data in real-time, enabling real-time monitoring of eBike performance and predictive maintenance.
Once potential failures have been identified, AI algorithms can provide recommendations for maintenance actions. For instance, if an eBike’s battery is showing signs of degradation, the AI system may recommend replacing the battery before it fails completely. This can help minimize downtime and ensure the eBike remains safe to use. Additionally, AI algorithms can provide recommendations for preventive maintenance tasks, such as regular cleaning and lubrication of the eBike’s chain and gears.
Predictive maintenance for eBikes using AI offers numerous benefits over traditional maintenance approaches. For instance, it can help reduce maintenance costs by minimizing downtime and preventing unnecessary maintenance tasks. Additionally, predictive maintenance can improve safety by ensuring the timely replacement of components before they fail. Furthermore, predictive maintenance can increase efficiency by optimizing maintenance schedules and reducing the need for regular maintenance tasks. By leveraging AI to analyze eBike sensor data, manufacturers and service providers can predict potential failures before they occur, minimizing downtime, reducing maintenance costs, and improving safety.
In summary, predictive maintenance for eBikes using AI is made possible through the analysis of data from eBike sensors. AI algorithms can identify patterns and anomalies in the data, predicting potential failures and providing recommendations for maintenance actions. This approach offers numerous benefits over traditional maintenance methods, including reduced maintenance costs, improved safety, and increased efficiency. By leveraging AI to analyze eBike sensor data, manufacturers and service providers can predict potential failures before they occur, minimizing downtime, reducing maintenance costs, and improving safety.
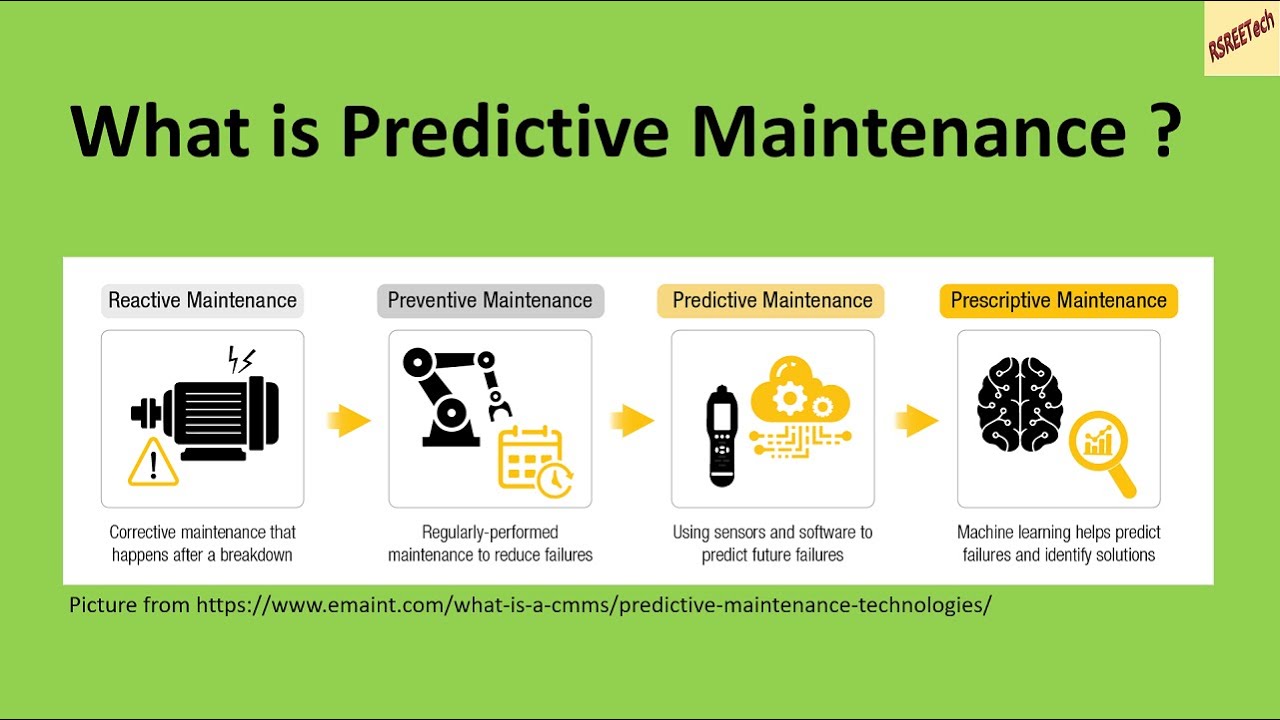
Real-World Applications of AI-Powered Predictive Maintenance for eBikes
Predictive maintenance for eBikes using AI is no longer a theoretical concept but a practical solution that has been successfully implemented by several eBike manufacturers and service providers. These companies have experienced numerous benefits, including reduced maintenance costs, increased customer satisfaction, and improved safety.
One example of a company that has successfully implemented AI-powered predictive maintenance is VanMoof, a Dutch eBike manufacturer. VanMoof has integrated AI-powered sensors into its eBikes that monitor various aspects of eBike performance, including battery health, motor temperature, and pedal assist levels. By analyzing this data, VanMoof can predict potential failures before they occur and proactively schedule maintenance tasks. This approach has helped VanMoof reduce maintenance costs and improve customer satisfaction by minimizing downtime.
Another example is Swiftmile, a California-based eBike sharing company. Swiftmile has implemented AI-powered predictive maintenance to monitor the health of its eBike fleets. By analyzing data from eBike sensors, Swiftmile can predict potential failures before they occur and schedule maintenance tasks accordingly. This approach has helped Swiftmile reduce maintenance costs, improve safety, and increase the longevity of its eBike components.
These examples demonstrate the potential benefits of AI-powered predictive maintenance for eBikes. By analyzing data from eBike sensors, AI algorithms can identify patterns and anomalies that may indicate potential failures before they occur. This approach can help reduce maintenance costs, improve safety, and increase the longevity of eBike components. Furthermore, by proactively scheduling maintenance tasks, eBike manufacturers and service providers can minimize downtime and improve customer satisfaction.
In summary, AI-powered predictive maintenance has been successfully implemented by several eBike manufacturers and service providers. These companies have experienced numerous benefits, including reduced maintenance costs, increased customer satisfaction, and improved safety. By analyzing data from eBike sensors, AI algorithms can identify potential failures before they occur, enabling proactive maintenance and minimizing downtime. As AI technology continues to evolve, we can expect to see even more innovative applications of predictive maintenance for eBikes.
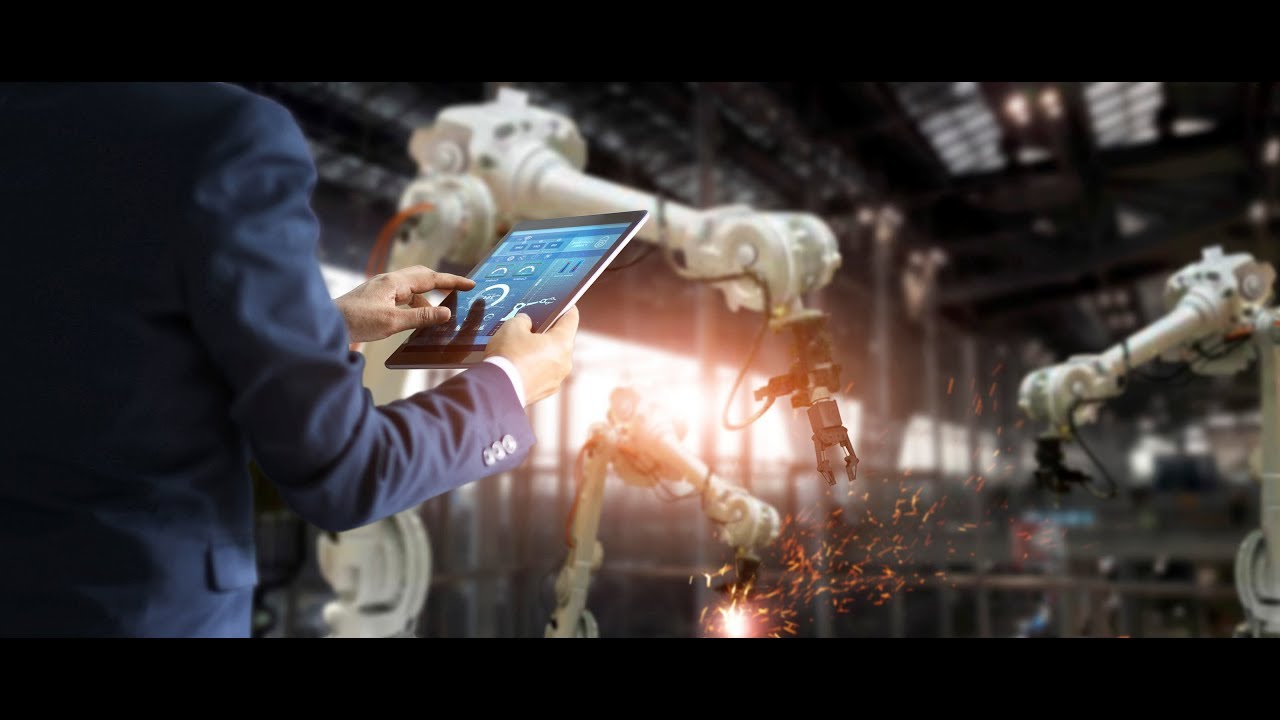
Selecting the Right AI Solution for Predictive Maintenance in eBikes
Predictive maintenance for eBikes using AI has the potential to revolutionize the eBike industry by minimizing downtime, reducing maintenance costs, and increasing safety and efficiency. However, selecting the right AI solution for predictive maintenance is crucial for realizing these benefits. Here are some key factors to consider when choosing an AI solution for predictive maintenance in eBikes:
- Data quality: The accuracy and completeness of the data collected from eBike sensors are crucial for the effectiveness of predictive maintenance. The AI solution should be able to handle data from various sources and formats, and it should be able to clean and preprocess the data to ensure accuracy.
- Scalability: As the number of eBikes in a fleet grows, the AI solution should be able to scale up to handle the increased volume of data. The solution should be able to process large amounts of data quickly and efficiently to provide real-time insights and predictions.
- Integration with existing systems: The AI solution should be able to integrate seamlessly with existing maintenance workflows and systems. This integration will help ensure that maintenance tasks are scheduled and executed efficiently and effectively.
- Cloud-based AI solutions: Cloud-based AI solutions offer several benefits, including scalability, flexibility, and cost-effectiveness. However, they also come with potential drawbacks, such as data privacy concerns and cybersecurity risks. It is essential to evaluate the security measures and data protection policies of the cloud provider before selecting a cloud-based AI solution.
By considering these factors, eBike manufacturers and service providers can select an AI solution that meets their specific needs and delivers the desired benefits. The right AI solution for predictive maintenance in eBikes can help reduce maintenance costs, improve safety and efficiency, and increase customer satisfaction.
In summary, selecting the right AI solution for predictive maintenance in eBikes is crucial for realizing the benefits of this innovative technology. Key factors to consider include data quality, scalability, integration with existing systems, and cloud-based solutions. By evaluating these factors and selecting an AI solution that meets their specific needs, eBike manufacturers and service providers can improve maintenance efficiency, reduce costs, and enhance safety and customer satisfaction.
Implementing AI-Powered Predictive Maintenance in eBike Operations
Implementing AI-powered predictive maintenance in eBike operations can help reduce maintenance costs, improve safety and efficiency, and increase customer satisfaction. Here is a step-by-step guide for implementing AI-powered predictive maintenance in eBike operations:
- Data collection: The first step in implementing AI-powered predictive maintenance is to collect data from eBike sensors, such as battery health, motor temperature, and pedal assist levels. This data can be collected using telematics devices, mobile apps, or other data collection methods.
- Data cleaning: Once the data has been collected, it is essential to clean and preprocess the data to ensure accuracy. This step involves removing any irrelevant or inaccurate data, filling in missing values, and normalizing the data.
- Data analysis: After cleaning the data, the next step is to analyze the data using AI algorithms. These algorithms can identify patterns and anomalies in the data, predict potential failures, and provide recommendations for maintenance actions. It is essential to choose the right AI algorithms for the specific needs of the eBike operation.
- Integration with existing systems: Once the AI solution has been developed, it is crucial to integrate it with existing maintenance workflows and systems. This integration will help ensure that maintenance tasks are scheduled and executed efficiently and effectively.
- Training staff: Finally, it is essential to train staff on using AI-powered tools and interpreting the results. This training will help ensure that maintenance tasks are carried out correctly and that the benefits of AI-powered predictive maintenance are fully realized.
By following these steps, eBike manufacturers and service providers can implement AI-powered predictive maintenance in their operations. However, it is essential to keep in mind that implementing AI-powered predictive maintenance is not a one-time process. It requires ongoing monitoring, evaluation, and optimization to ensure that it continues to deliver the desired benefits.
In summary, implementing AI-powered predictive maintenance in eBike operations involves collecting and cleaning data, analyzing the data using AI algorithms, integrating the AI solution with existing systems, and training staff on using AI-powered tools. By following these steps, eBike manufacturers and service providers can reduce maintenance costs, improve safety and efficiency, and increase customer satisfaction. However, it is essential to keep in mind that implementing AI-powered predictive maintenance is an ongoing process that requires ongoing monitoring, evaluation, and optimization.
Overcoming Challenges in AI-Powered Predictive Maintenance for eBikes
Implementing AI-powered predictive maintenance in eBike operations can provide numerous benefits, such as reduced maintenance costs, increased safety, and improved efficiency. However, there are several challenges that eBike manufacturers and service providers may face when implementing these advanced maintenance strategies. By understanding and addressing these challenges, organizations can ensure the successful implementation of predictive maintenance for eBikes using AI.
Data Privacy Concerns
One of the primary challenges in implementing AI-powered predictive maintenance for eBikes is addressing data privacy concerns. As eBikes generate vast amounts of data, it is crucial to ensure that this data is collected, stored, and processed in compliance with relevant data protection regulations, such as the General Data Protection Regulation (GDPR) in the European Union. To address these concerns, eBike manufacturers and service providers should implement robust data protection policies and practices, ensuring that data is collected and processed with the explicit consent of users and is used solely for the purpose of predictive maintenance.
Cybersecurity Risks
Another challenge in implementing AI-powered predictive maintenance for eBikes is addressing cybersecurity risks. As eBikes become increasingly connected, they become vulnerable to cyber attacks, which can compromise the confidentiality, integrity, and availability of data. To mitigate these risks, eBike manufacturers and service providers should implement robust cybersecurity measures, such as encryption, firewalls, and intrusion detection systems, to protect against unauthorized access and ensure the confidentiality, integrity, and availability of data.
Regulatory Compliance
Regulatory compliance is another challenge in implementing AI-powered predictive maintenance for eBikes. As eBikes become increasingly advanced, they are subject to a growing number of regulations, such as safety standards and emissions regulations. To ensure compliance with these regulations, eBike manufacturers and service providers should stay up-to-date with the latest regulatory requirements and implement robust compliance policies and practices. This can include conducting regular compliance audits, implementing compliance training programs, and maintaining accurate records of compliance activities.
Recommendations for Addressing Challenges
To address these challenges and ensure the successful implementation of predictive maintenance for eBikes using AI, eBike manufacturers and service providers should consider the following recommendations:
- Implement robust data protection policies and practices to address data privacy concerns.
- Implement robust cybersecurity measures to protect against cyber attacks and ensure the confidentiality, integrity, and availability of data.
- Stay up-to-date with the latest regulatory requirements and implement robust compliance policies and practices.
- Collaborate with AI solution providers and cybersecurity experts to ensure the successful implementation of predictive maintenance for eBikes using AI.
- Invest in ongoing research and development to stay at the forefront of AI-powered predictive maintenance for eBikes and ensure the long-term success of eBike operations.
By addressing these challenges and implementing AI-powered predictive maintenance for eBikes, organizations can realize numerous benefits, such as reduced maintenance costs, increased safety, and improved efficiency. With the right approach, AI-powered predictive maintenance can revolutionize eBike maintenance and provide a competitive advantage in the eBike industry.
The Future of Predictive Maintenance for eBikes using AI
Predictive maintenance for eBikes using AI has the potential to revolutionize the eBike industry, providing numerous benefits for eBike manufacturers, service providers, and riders alike. As AI technology continues to advance, we can expect to see several exciting developments in predictive maintenance for eBikes in the coming years.
Real-Time Monitoring
One of the most significant developments in predictive maintenance for eBikes using AI is real-time monitoring. With the help of advanced sensors and AI algorithms, eBike manufacturers and service providers can monitor the health and performance of eBike components in real-time, detecting potential failures before they occur and taking proactive maintenance actions. Real-time monitoring can help to minimize downtime, reduce maintenance costs, and improve the overall safety and reliability of eBikes.
Autonomous Maintenance
Another exciting development in predictive maintenance for eBikes using AI is autonomous maintenance. With the help of advanced robotics and AI algorithms, eBike manufacturers and service providers can automate routine maintenance tasks, such as cleaning, lubrication, and adjustments. Autonomous maintenance can help to reduce maintenance costs, improve efficiency, and ensure the consistent quality of maintenance services.
Predictive Analytics for eBike Fleets
Predictive analytics for eBike fleets is another area where we can expect to see significant developments in the coming years. With the help of AI algorithms, eBike fleet operators can analyze data from multiple eBikes in real-time, identifying trends and patterns that can help to optimize fleet performance, reduce maintenance costs, and improve safety. Predictive analytics for eBike fleets can help to improve the overall efficiency and profitability of eBike sharing and rental services.
Benefits of AI-Powered Predictive Maintenance for eBikes
The benefits of AI-powered predictive maintenance for eBikes are numerous, including reduced maintenance costs, increased safety, improved efficiency, and longer component lifespans. By leveraging the power of AI, eBike manufacturers and service providers can provide more reliable, cost-effective, and sustainable maintenance services, improving the overall customer experience and building brand loyalty.
Potential Drawbacks of AI-Powered Predictive Maintenance for eBikes
While the benefits of AI-powered predictive maintenance for eBikes are significant, there are also potential drawbacks to consider. These include the high cost of implementing AI solutions, the need for specialized expertise to manage and maintain AI systems, and the potential for false positives or false negatives in predictive maintenance algorithms. It is essential for eBike manufacturers and service providers to carefully evaluate the costs and benefits of AI-powered predictive maintenance and to implement robust quality control measures to ensure the accuracy and reliability of predictive maintenance algorithms.
In conclusion, predictive maintenance for eBikes using AI has the potential to revolutionize the eBike industry, providing numerous benefits for manufacturers, service providers, and riders alike. As AI technology continues to advance, we can expect to see exciting developments in real-time monitoring, autonomous maintenance, and predictive analytics for eBike fleets. By carefully evaluating the costs and benefits of AI-powered predictive maintenance and implementing robust quality control measures, eBike manufacturers and service providers can leverage the power of AI to provide more reliable, cost-effective, and sustainable maintenance services, improving the overall customer experience and building brand loyalty.