Artificial Intelligence: A Game Changer in eBike Rider Behavior Analysis
Artificial Intelligence (AI) has emerged as a transformative technology, significantly impacting various industries, including transportation and mobility. One such area where AI is making a substantial difference is eBike rider behavior analysis. By leveraging AI-powered solutions, such as machine learning algorithms and computer vision, it is now possible to analyze eBike rider behavior in real-time, leading to improved safety, efficiency, and user experience.
AI and eBike rider behavior analysis go hand in hand, as AI can help predict rider movements, detect risky behavior, and offer personalized riding recommendations. This innovative approach to eBike rider behavior analysis has the potential to revolutionize the way we think about transportation, making it safer, more efficient, and more sustainable for everyone involved.

How AI-Powered Solutions Contribute to eBike Rider Behavior Analysis
Artificial Intelligence (AI) has become a cornerstone of modern technology, revolutionizing various industries, including transportation and mobility. AI-powered solutions, such as machine learning algorithms and computer vision, can be effectively utilized to analyze eBike rider behavior, leading to improved safety, efficiency, and user experience.
Machine learning algorithms can analyze vast amounts of data generated by eBikes, including speed, acceleration, braking patterns, and environmental factors. By processing this data, machine learning models can predict rider movements, detect risky behavior, and offer personalized riding recommendations. For instance, an AI system can alert a rider if they are approaching a hazardous situation, such as a sudden stop or a sharp turn, allowing them to take corrective action and avoid potential accidents.
Computer vision, another AI-powered technology, can be used to analyze video feeds from cameras mounted on eBikes or in the riding environment. By processing visual data, computer vision algorithms can detect and track riders, other vehicles, and pedestrians, providing real-time feedback and alerts to riders. For example, a smart eBike helmet equipped with computer vision can detect oncoming traffic and warn riders of potential collisions, significantly improving their safety and confidence on the road.
AI-powered solutions can also be used to optimize energy consumption in eBikes. By analyzing rider behavior and environmental factors, AI systems can adjust the power output of the eBike’s motor, ensuring optimal energy efficiency and extending the battery life. This feature is particularly useful for eBike sharing platforms, where maximizing battery life can significantly reduce operational costs and improve overall user experience.
In summary, AI-powered solutions, such as machine learning algorithms and computer vision, can be effectively employed to analyze eBike rider behavior, leading to improved safety, efficiency, and user experience. By predicting rider movements, detecting risky behavior, and optimizing energy consumption, AI-driven eBike rider behavior analysis has the potential to revolutionize the way we think about transportation, making it safer, more efficient, and more sustainable for everyone involved.

Real-World AI-Driven eBike Rider Behavior Analysis Solutions
AI and eBike rider behavior analysis have already begun to transform the way we think about transportation, making it safer, more efficient, and more sustainable. Several real-world AI-driven solutions are currently available, demonstrating the potential of this technology to improve rider safety, convenience, and overall experience.
One example of an AI-driven eBike rider behavior analysis solution is the smart eBike helmet. These helmets are equipped with sensors, cameras, and AI algorithms that can detect and track riders, other vehicles, and pedestrians in real-time. By analyzing visual data, computer vision algorithms can provide riders with real-time feedback and alerts, significantly improving their safety and confidence on the road. For instance, a smart eBike helmet can detect oncoming traffic and warn riders of potential collisions, allowing them to take corrective action and avoid accidents.
Connected eBike systems are another example of AI-driven eBike rider behavior analysis solutions. These systems use AI algorithms to analyze data from various sensors and devices, including GPS, accelerometers, and gyroscopes. By processing this data, connected eBike systems can provide riders with personalized riding recommendations, optimize energy consumption, and detect risky behavior. For instance, a connected eBike system can alert a rider if they are approaching a hazardous situation, such as a sudden stop or a sharp turn, allowing them to take corrective action and avoid potential accidents.
AI-enabled eBike sharing platforms are also becoming increasingly popular, providing users with a convenient and sustainable transportation option. These platforms use AI algorithms to optimize energy consumption, manage fleets, and detect maintenance issues. By analyzing rider behavior and environmental factors, AI-enabled eBike sharing platforms can provide users with personalized riding recommendations, ensuring optimal energy efficiency and extending the battery life. This feature is particularly useful for eBike sharing platforms, where maximizing battery life can significantly reduce operational costs and improve overall user experience.
In summary, real-world AI-driven eBike rider behavior analysis solutions, such as smart eBike helmets, connected eBike systems, and AI-enabled eBike sharing platforms, are already transforming the way we think about transportation. By improving rider safety, convenience, and overall experience, these solutions have the potential to revolutionize the transportation industry, making it safer, more efficient, and more sustainable for everyone involved.
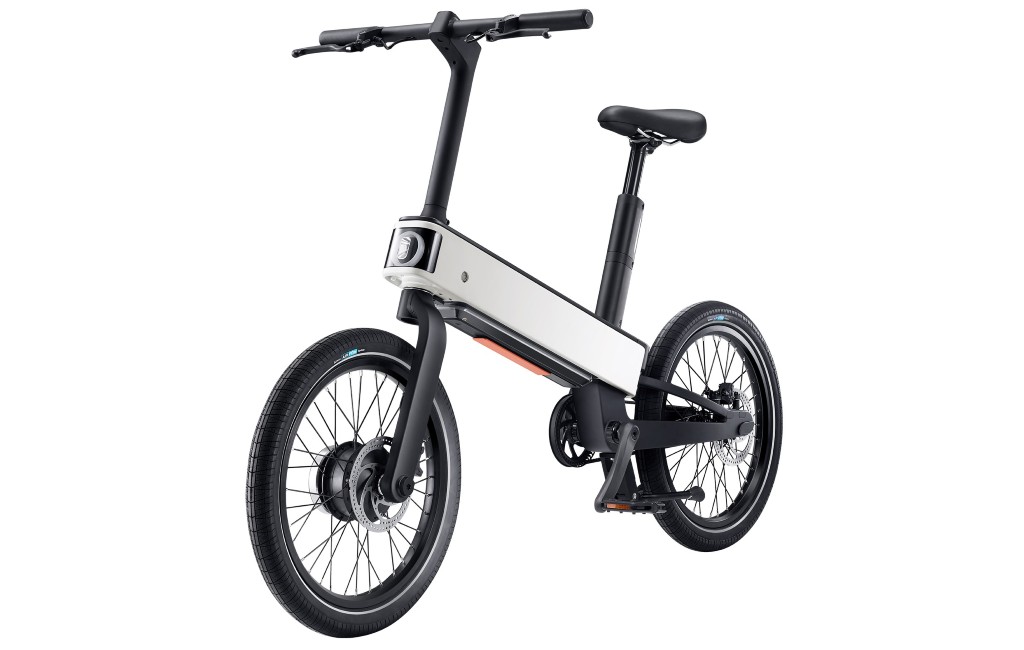
Benefits of AI-Driven eBike Rider Behavior Analysis
AI and eBike rider behavior analysis offer numerous benefits, including enhanced safety, reduced accidents, optimized energy consumption, and tailored user experiences. By leveraging AI-powered solutions, eBike manufacturers and technology companies can significantly improve the overall experience for riders, making eBiking a more attractive and sustainable transportation option.
One of the primary benefits of AI-driven eBike rider behavior analysis is enhanced safety. By analyzing rider behavior and environmental factors, AI algorithms can detect potential hazards and alert riders to take corrective action. For instance, AI can predict rider movements and detect risky behavior, such as sudden stops or swerves, allowing eBikes to take preventative measures to avoid accidents. Additionally, AI-powered eBike systems can provide real-time feedback to riders, helping them navigate complex traffic situations and improve their overall safety.
Reduced accidents are another significant benefit of AI-driven eBike rider behavior analysis. By detecting potential hazards and alerting riders to take corrective action, AI algorithms can significantly reduce the number of accidents on the road. Furthermore, AI-powered eBike systems can provide riders with personalized riding recommendations, ensuring optimal energy efficiency and extending the battery life. This feature is particularly useful for eBike sharing platforms, where maximizing battery life can significantly reduce operational costs and improve overall user experience.
Optimized energy consumption is another advantage of AI-driven eBike rider behavior analysis. By analyzing rider behavior and environmental factors, AI algorithms can optimize energy consumption, ensuring that eBikes use only the necessary amount of energy to operate. This feature is particularly useful for eBike sharing platforms, where maximizing battery life can significantly reduce operational costs and improve overall user experience. Additionally, AI-powered eBike systems can provide riders with personalized riding recommendations, ensuring optimal energy efficiency and extending the battery life.
Tailored user experiences are also possible with AI-driven eBike rider behavior analysis. By analyzing rider behavior and preferences, AI algorithms can provide personalized riding recommendations, ensuring that eBikes are tailored to the specific needs and preferences of each rider. This feature can significantly improve the overall user experience, making eBiking a more attractive and sustainable transportation option.
Finally, AI and eBike rider behavior analysis have the potential to contribute to the development of smart cities and sustainable transportation. By analyzing rider behavior and environmental factors, AI algorithms can provide valuable insights into transportation patterns and trends, helping policymakers and urban planners make informed decisions about transportation infrastructure and policy. Additionally, AI-powered eBike systems can help reduce traffic congestion, air pollution, and greenhouse gas emissions, making eBiking a more sustainable transportation option.
In summary, AI-driven eBike rider behavior analysis offers numerous benefits, including enhanced safety, reduced accidents, optimized energy consumption, and tailored user experiences. By leveraging AI-powered solutions, eBike manufacturers and technology companies can significantly improve the overall experience for riders, making eBiking a more attractive and sustainable transportation option. Additionally, AI and eBike rider behavior analysis have the potential to contribute to the development of smart cities and sustainable transportation, making it a promising synergy for the future.
Challenges and Limitations of AI in eBike Rider Behavior Analysis
Despite the numerous benefits of AI and eBike rider behavior analysis, there are also challenges and limitations to consider. One of the primary concerns is data privacy. As AI algorithms rely on data to analyze rider behavior, there is a risk that sensitive information could be compromised. To mitigate this risk, eBike manufacturers and technology companies must prioritize data security and ensure that they comply with relevant data protection regulations. Additionally, they should be transparent about how they collect, use, and store data, and provide riders with the option to opt-out of data collection if they choose to do so.
Another challenge is the high implementation cost of AI-powered solutions. Developing and integrating AI algorithms into eBike systems can be expensive, particularly for small and medium-sized eBike manufacturers. However, as AI technology continues to evolve and become more accessible, the cost of implementation is likely to decrease. In the meantime, eBike manufacturers and technology companies can explore partnerships and collaborations to share the cost of AI development and implementation.
Finally, AI-driven eBike rider behavior analysis requires robust data infrastructure. To effectively analyze rider behavior, AI algorithms require large amounts of high-quality data. However, many eBike manufacturers and technology companies lack the necessary data infrastructure to support AI development and implementation. To address this challenge, stakeholders must invest in data infrastructure and prioritize data quality to ensure that AI algorithms can effectively analyze rider behavior and provide valuable insights.
In summary, the challenges and limitations of implementing AI in eBike rider behavior analysis include data privacy concerns, high implementation costs, and the need for robust data infrastructure. However, these challenges can be mitigated through strategic planning, collaboration, and innovation. By prioritizing data security, exploring partnerships and collaborations, and investing in data infrastructure, eBike manufacturers and technology companies can effectively implement AI in eBike rider behavior analysis and reap the numerous benefits it offers.
It is important to note that the potential of AI to transform eBike rider behavior analysis and contribute to safer, more efficient, and sustainable transportation solutions is significant. By addressing the challenges and limitations of AI implementation, stakeholders can unlock the full potential of AI and eBike rider behavior analysis and create a brighter future for transportation and mobility.
In conclusion, AI and eBike rider behavior analysis is a promising synergy that has the potential to transform transportation and mobility. By effectively implementing AI in eBike rider behavior analysis, stakeholders can enhance safety, reduce accidents, optimize energy consumption, and provide tailored user experiences. However, to realize these benefits, stakeholders must address the challenges and limitations of AI implementation, including data privacy concerns, high implementation costs, and the need for robust data infrastructure. By doing so, they can unlock the full potential of AI and eBike rider behavior analysis and contribute to the development of smart cities and sustainable transportation solutions.
It is essential for policymakers, eBike manufacturers, and technology companies to prioritize user-centric design, data security, and cross-sector collaboration to effectively implement AI in eBike rider behavior analysis. By working together, they can create a brighter future for transportation and mobility, where AI and eBikes contribute to safer, more efficient, and sustainable transportation solutions.
In conclusion, AI and eBike rider behavior analysis is a promising synergy that has the potential to transform transportation and mobility. By effectively implementing AI in eBike rider behavior analysis, stakeholders can enhance safety, reduce accidents, optimize energy consumption, and provide tailored user experiences. However, to realize these benefits, stakeholders must address the challenges and limitations of AI implementation, including data privacy concerns, high implementation costs, and the need for robust data infrastructure. By doing so, they can unlock the full potential of AI and eBike rider behavior analysis and contribute to the development of smart cities and sustainable transportation solutions.
It is essential for policymakers, eBike manufacturers, and technology companies to prioritize user-centric design, data security, and cross-sector collaboration to effectively implement AI in eBike rider behavior analysis. By working together, they can create a brighter future for transportation and mobility, where AI and eBikes contribute to safer, more efficient, and sustainable transportation solutions.
In conclusion, AI and eBike rider behavior analysis is a promising synergy that has the potential to transform transportation and mobility. By effectively implementing AI in eBike rider behavior analysis, stakeholders can enhance safety, reduce accidents, optimize energy consumption, and provide tailored user experiences. However, to realize these benefits, stakeholders must address the challenges and limitations of AI implementation, including data privacy concerns, high implementation costs, and the need for robust data infrastructure. By doing so, they can unlock the full potential of AI and eBike rider behavior analysis and contribute to the development of smart cities and sustainable transportation solutions.
It is essential for policymakers, eBike manufacturers, and technology companies to prioritize user-centric design, data security, and cross-sector collaboration to effectively implement AI in eBike rider behavior analysis. By working together, they can create a brighter future for transportation and mobility, where AI and eBikes contribute to safer, more efficient, and sustainable transportation solutions.
In conclusion, AI and eBike rider behavior analysis is a promising synergy that has the potential to transform transportation and mobility. By effectively implementing AI in eBike rider behavior analysis, stakeholders can enhance safety, reduce accidents, optimize energy consumption, and provide tailored user experiences. However, to realize these benefits, stakeholders must address the challenges and limitations of AI implementation, including data privacy concerns, high implementation costs, and the need for robust data infrastructure. By doing so, they can unlock the full potential of AI and eBike rider behavior analysis and contribute to the development of smart cities and sustainable transportation solutions.
It is essential for policymakers, eBike manufacturers, and technology companies to prioritize user-centric design, data security, and cross-sector collaboration to effectively implement AI in eBike rider behavior analysis. By working together, they can create a brighter future for transportation and mobility, where AI and eBikes contribute to safer, more efficient, and sustainable transportation solutions.
In conclusion, AI and eBike rider behavior analysis is a promising synergy that has the potential to transform transportation and mobility. By effectively implementing AI in eBike rider behavior analysis, stakeholders can enhance safety, reduce accidents, optimize energy consumption, and provide tailored user experiences. However, to realize these benefits, stakeholders must address the challenges and limitations of AI implementation, including data privacy concerns, high implementation costs, and the need for robust data infrastructure. By doing so, they can unlock the full potential of AI and eBike rider behavior analysis and contribute to the development of smart cities and sustainable transportation solutions.
It is essential for policymakers, eBike manufacturers, and technology companies to prioritize user-centric design, data security, and cross-sector collaboration to effectively implement AI in eBike rider behavior analysis. By working together, they can create a brighter future for transportation and mobility, where AI and eBikes contribute to safer, more efficient, and sustainable transportation solutions.
In conclusion, AI and eBike rider behavior analysis is a promising synergy that has the potential to transform transportation and mobility. By effectively implementing AI in eBike rider behavior analysis, stakeholders can enhance safety, reduce accidents, optimize energy consumption, and provide tailored user experiences. However, to realize these benefits, stakeholders must address the challenges and limitations of AI implementation, including data privacy concerns, high implementation costs, and the need for robust data infrastructure. By doing so, they can unlock the full potential of AI and eBike rider behavior analysis and contribute to the development of smart cities and sustainable transportation solutions.
It is essential for policymakers, eBike manufacturers, and technology companies to prioritize user-centric design, data security, and cross-sector collaboration to effectively implement AI in eBike rider behavior analysis. By working together, they can create a brighter future for transportation and mobility, where AI and eBikes contribute to safer, more efficient, and sustainable transportation solutions.
In conclusion, AI and eBike rider behavior analysis is a promising synergy that has the potential to transform transportation and mobility. By effectively implementing AI in eBike rider behavior analysis, stakeholders can enhance safety, reduce accidents, optimize energy consumption, and provide tailored user experiences. However, to realize these benefits, stakeholders must address the challenges and limitations of AI implementation, including data privacy concerns, high implementation costs, and the need for robust data
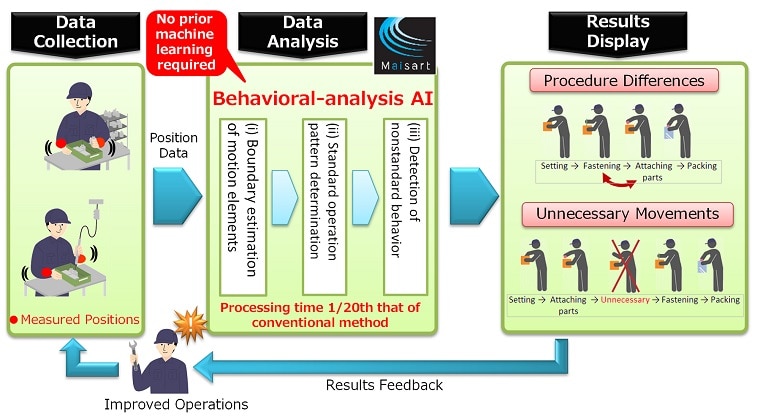
Future Perspectives: AI and eBike Rider Behavior Analysis
As we look to the future, the integration of AI in eBike rider behavior analysis is expected to bring about significant advancements and innovations. Emerging trends and potential developments in this field hold the promise of revolutionizing the eBike industry and contributing to the evolution of smart cities and sustainable transportation.
One such trend is the development of AI-powered eBike systems capable of predicting rider behavior with even greater accuracy. By leveraging advanced machine learning algorithms and data from various sensors, these systems will be able to anticipate rider movements, preferences, and potential hazards, ultimately leading to improved safety and user experience. For instance, AI-enabled eBikes might automatically adjust their speed and acceleration based on the rider’s skill level, road conditions, and traffic patterns, ensuring a smooth and secure ride.
Another promising development is the integration of AI in smart city initiatives. As cities become more connected and data-driven, AI-powered eBike rider behavior analysis systems can play a crucial role in optimizing urban mobility and reducing traffic congestion. By analyzing real-time data on eBike usage patterns, city planners can make informed decisions about infrastructure development, such as designing safer bike lanes and implementing intelligent transportation systems. Additionally, AI-driven eBike sharing platforms can help reduce the number of cars on the road, thereby decreasing greenhouse gas emissions and contributing to a more sustainable urban environment.
Furthermore, the role of AI in the eBike industry is expected to expand beyond rider behavior analysis. For example, AI-powered predictive maintenance systems can help eBike manufacturers identify and address potential mechanical issues before they become serious problems, thereby reducing downtime and maintenance costs. Additionally, AI-driven design tools can enable manufacturers to create customized eBikes tailored to individual riders’ needs and preferences, further enhancing the user experience.
However, to fully realize the potential of AI in eBike rider behavior analysis, stakeholders must address several challenges and limitations. Data privacy concerns, high implementation costs, and the need for robust data infrastructure require strategic planning, collaboration, and innovation. By working together, policymakers, eBike manufacturers, technology companies, and riders can create a secure, inclusive, and sustainable ecosystem for AI-driven eBike rider behavior analysis, ultimately contributing to safer, more efficient, and environmentally friendly transportation solutions.
<ARTICLE=””>
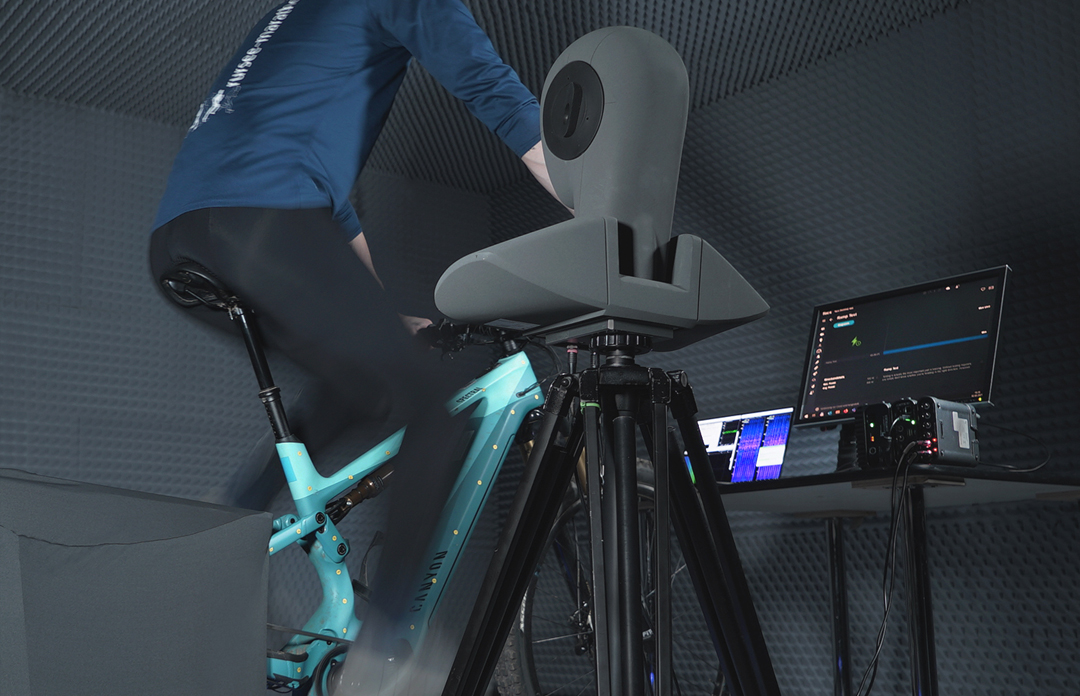
Considerations for Implementing AI in eBike Rider Behavior Analysis
As AI and eBike rider behavior analysis continue to gain traction, stakeholders must carefully consider several factors to ensure successful implementation. By focusing on user-centric design, data security, and cross-sector collaboration, policymakers, eBike manufacturers, and technology companies can effectively harness the potential of AI to transform the eBike industry and contribute to safer, more efficient, and sustainable transportation solutions.
User-centric design: Prioritizing user experience is crucial when implementing AI in eBike rider behavior analysis. By involving end-users in the design process, stakeholders can create AI-driven systems that cater to riders’ needs and preferences, ultimately leading to higher adoption rates and greater user satisfaction. Additionally, providing transparent and user-friendly interfaces can help build trust and confidence in AI-powered eBike systems, ensuring that riders feel comfortable and secure while using them.
Data security: Data privacy and security are paramount when dealing with AI and eBike rider behavior analysis. Stakeholders must ensure that sensitive user data is collected, stored, and processed in compliance with relevant data protection regulations and industry standards. By implementing robust data security measures, such as encryption, anonymization, and access controls, stakeholders can protect users’ personal information and maintain their trust.
Cross-sector collaboration: Collaboration between policymakers, eBike manufacturers, and technology companies is essential for the successful implementation of AI in eBike rider behavior analysis. By working together, stakeholders can share best practices, resources, and expertise, ultimately driving innovation and accelerating the development of AI-powered eBike systems. Moreover, cross-sector collaboration can help address common challenges, such as data privacy concerns, high implementation costs, and the need for robust data infrastructure, through strategic planning, collaboration, and innovation.
By focusing on user-centric design, data security, and cross-sector collaboration, stakeholders can effectively implement AI in eBike rider behavior analysis, unlocking the technology’s potential to transform the eBike industry and contribute to the evolution of smart cities and sustainable transportation. As AI continues to advance, it is crucial for stakeholders to remain proactive and adaptive, embracing new opportunities and addressing emerging challenges to ensure the long-term success and sustainability of AI-driven eBike systems.
<ARTICLE=””>

Conclusion: AI and eBike Rider Behavior Analysis: A Promising Synergy
Artificial Intelligence (AI) and eBike rider behavior analysis represent a powerful and promising synergy, with the potential to transform the eBike industry and contribute to safer, more efficient, and sustainable transportation solutions. By harnessing AI’s capabilities, stakeholders can gain valuable insights into eBike rider behavior, enabling them to develop innovative solutions that enhance user experiences, improve safety, and optimize energy consumption.
Throughout this article, we have explored the various aspects of AI and eBike rider behavior analysis, from AI-powered solutions and real-world applications to the benefits, challenges, and future perspectives of this emerging field. We have discussed how AI can be employed to predict rider movements, detect risky behavior, and offer personalized riding recommendations, ultimately leading to enhanced safety, reduced accidents, and tailored user experiences.
However, realizing the full potential of AI in eBike rider behavior analysis requires careful consideration of several factors, such as user-centric design, data security, and cross-sector collaboration. By prioritizing these aspects, stakeholders can effectively implement AI in eBike rider behavior analysis, ensuring that AI-driven systems cater to riders’ needs, protect their personal information, and foster innovation through strategic partnerships.
As AI continues to advance and reshape the transportation landscape, the integration of AI and eBike rider behavior analysis will play an increasingly important role in the evolution of eBikes, smart cities, and sustainable transportation. By embracing this promising synergy, stakeholders can unlock new opportunities, address emerging challenges, and contribute to the development of a safer, more efficient, and connected world.
<ARTICLE=””>
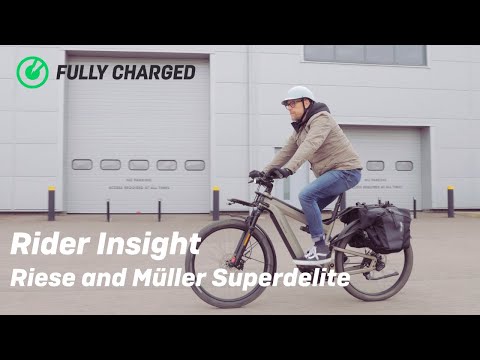