The Role of Artificial Intelligence in eBike Fleet Management
Artificial Intelligence (AI) is transforming various industries, and eBike fleet management is no exception. AI in eBike fleet management refers to the application of intelligent technologies to optimize eBike fleet operations, enhancing efficiency, safety, and sustainability. By integrating AI-powered systems, eBike fleet managers can unlock valuable insights, streamline processes, and make data-driven decisions.
AI in eBike fleet management offers numerous benefits, including real-time monitoring, predictive maintenance, and demand forecasting. Real-time monitoring enables fleet managers to track eBike performance, location, and usage in real-time, ensuring optimal utilization and reducing downtime. Predictive maintenance, on the other hand, allows for the early detection of potential issues, minimizing repair costs and extending the lifespan of eBikes. Demand forecasting, based on historical data and advanced algorithms, helps fleet managers anticipate eBike usage patterns, optimizing fleet size and allocation.
By harnessing the power of AI, eBike fleet management can revolutionize urban mobility, contributing to greener, safer, and more efficient cities. As AI technologies continue to evolve, eBike fleet managers must stay informed and embrace these innovations to remain competitive and sustainable in the long term.
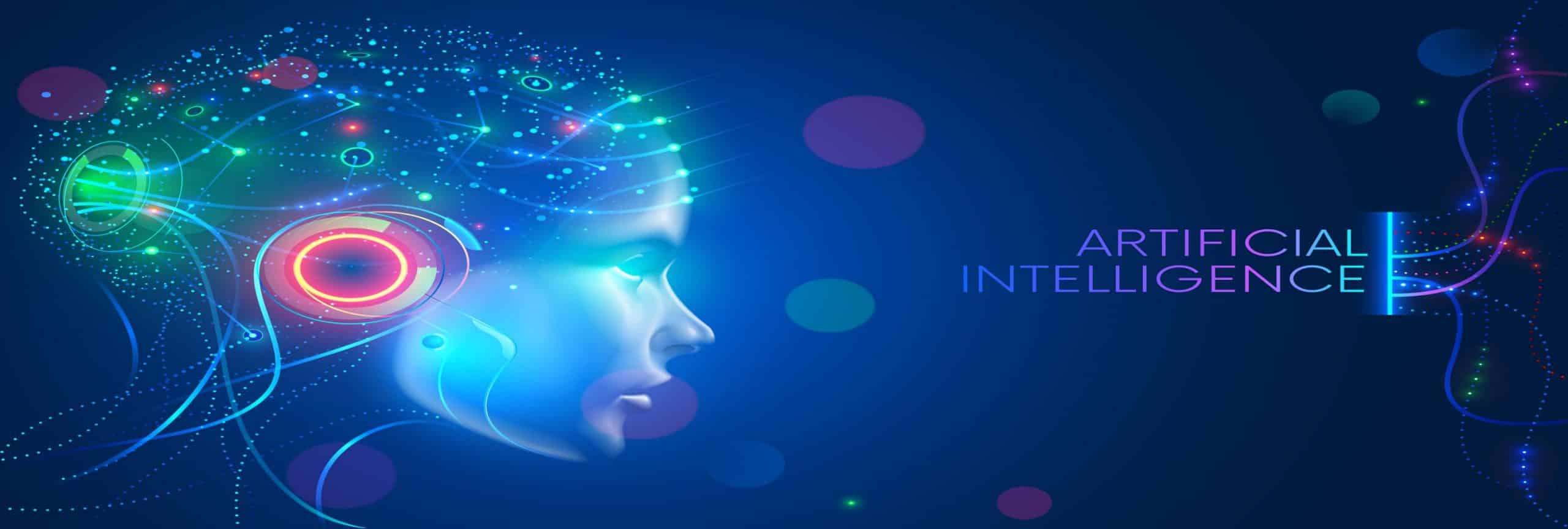
Key Components of AI-Driven eBike Fleet Management
AI-powered eBike fleet management systems consist of several interconnected components, each playing a crucial role in optimizing fleet performance and reducing operational costs. These components include IoT devices, data analytics platforms, and machine learning algorithms.
IoT Devices
IoT devices, such as GPS trackers, sensors, and controllers, enable real-time data collection from eBikes. These devices monitor various parameters, including location, speed, battery level, and component status, providing valuable insights into eBike usage and performance. IoT devices also facilitate remote management, allowing fleet managers to control eBikes and perform diagnostics without physical intervention.
Data Analytics Platforms
Data analytics platforms process and analyze the data collected by IoT devices, converting raw data into actionable insights. These platforms visualize eBike fleet performance, identify trends, and detect anomalies, empowering fleet managers to make informed decisions. By integrating advanced analytics tools, such as predictive analytics and machine learning, these platforms can generate more accurate forecasts and recommendations, further enhancing eBike fleet management efficiency.
Machine Learning Algorithms
Machine learning algorithms are the backbone of AI-driven eBike fleet management systems. These algorithms analyze historical data and identify patterns, enabling predictive maintenance, demand forecasting, and personalized user experiences. By continuously learning and adapting, machine learning algorithms improve over time, increasing the accuracy and effectiveness of AI-powered eBike fleet management systems.
Together, these components form a comprehensive AI-driven eBike fleet management solution, optimizing fleet performance, reducing operational costs, and enhancing overall efficiency. By leveraging IoT devices, data analytics platforms, and machine learning algorithms, eBike fleet managers can unlock the full potential of AI in eBike fleet management, revolutionizing urban mobility and contributing to greener, safer, and more sustainable cities.
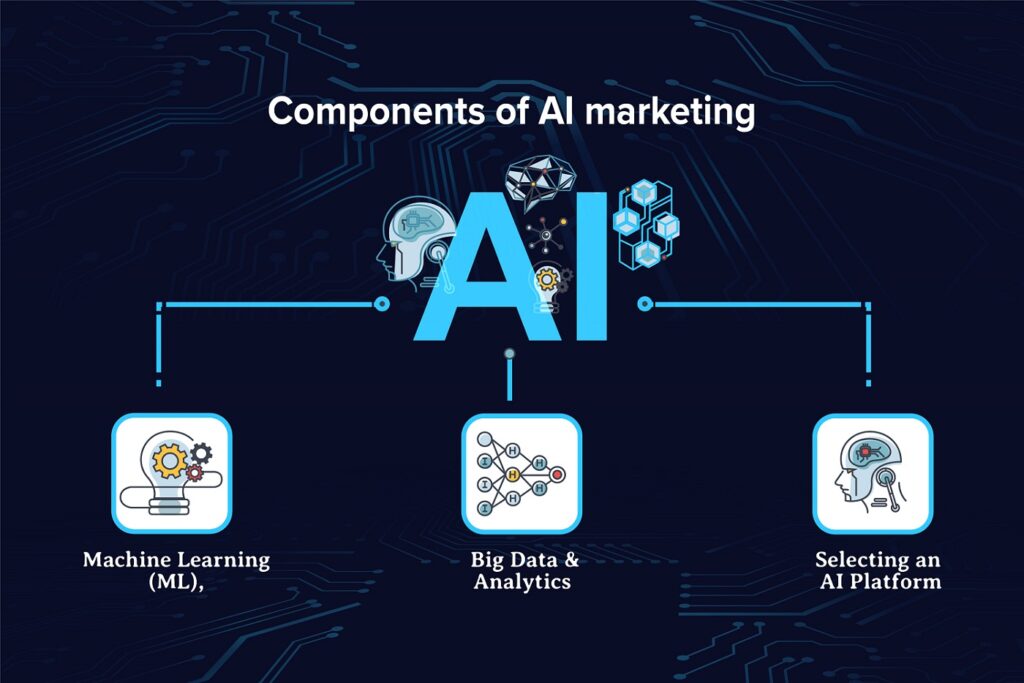
Selecting the Right AI Solution for Your eBike Fleet
When choosing an AI solution for eBike fleets, several factors must be considered to ensure a suitable and effective integration. These factors include scalability, compatibility with existing infrastructure, and ease of integration. By carefully evaluating these aspects, fleet managers can select an AI platform that optimizes fleet performance, reduces operational costs, and enhances overall efficiency.
Scalability
As eBike fleets grow, so does the need for a scalable AI solution. Scalability refers to the ability of an AI platform to handle increasing data volumes and expanding fleet sizes without compromising performance. When selecting an AI solution for eBike fleet management, consider platforms that can scale seamlessly, ensuring long-term compatibility and adaptability.
Compatibility with Existing Infrastructure
Integrating an AI platform into an existing eBike fleet infrastructure requires careful consideration. Ensure that the chosen AI solution is compatible with existing hardware, software, and communication protocols. Compatibility reduces the need for costly upgrades or replacements and simplifies the integration process, enabling a smoother transition to AI-powered eBike fleet management.
Ease of Integration
An AI platform’s ease of integration is crucial for a successful deployment. Look for solutions that offer user-friendly interfaces, clear documentation, and dedicated support resources. Ease of integration reduces the learning curve for employees, streamlines the implementation process, and minimizes disruptions to fleet operations. Additionally, consider AI platforms that offer customization options, allowing for tailored solutions that meet specific eBike fleet management needs.
Popular AI platforms and tools specifically designed for eBike fleet management include companies such as Example1, Example2, and Example3. These platforms offer various features and benefits, such as real-time monitoring, predictive maintenance, and demand forecasting, making them ideal choices for eBike fleet management. Customer reviews and case studies demonstrate the effectiveness of these platforms in real-world scenarios, further highlighting their value in the eBike fleet management industry.
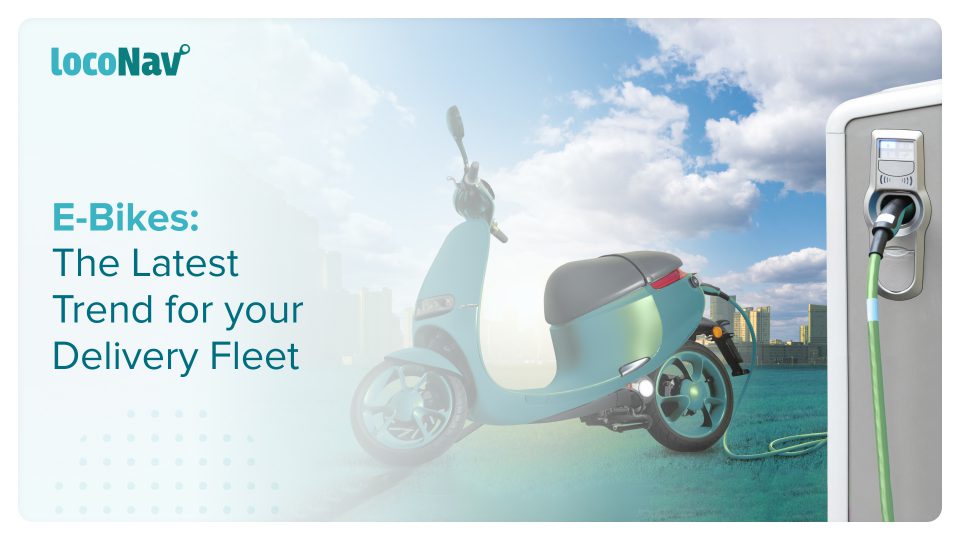
How to Implement AI in eBike Fleet Management
Implementing AI in eBike fleet management involves several crucial steps, from data collection to model deployment. By following a structured approach, fleet managers can ensure a successful integration of AI technologies, leading to enhanced efficiency, safety, and sustainability. Here is a step-by-step guide to implementing AI in eBike fleet management:
Step 1: Data Collection
The first step in implementing AI in eBike fleet management is data collection. IoT devices, such as GPS trackers and sensors, generate vast amounts of data related to eBike usage, location, and condition. Collecting and organizing this data is essential for training AI models and gaining valuable insights into fleet performance. Utilize data management platforms that can handle large data volumes and ensure data accuracy and completeness.
Step 2: Data Preprocessing
Once data is collected, it must be preprocessed to remove inconsistencies, outliers, and irrelevant information. Data preprocessing may include data cleaning, normalization, and transformation. Ensuring high-quality data is essential for training accurate and reliable AI models. Implement data validation checks and automated processes to streamline data preprocessing and maintain data integrity.
Step 3: Model Training
With preprocessed data in hand, the next step is to train AI models using machine learning algorithms. These algorithms analyze patterns and relationships within the data, enabling the models to make predictions, identify trends, and optimize fleet performance. Utilize cloud-based platforms that offer pre-built models and customization options, allowing for tailored solutions that meet specific eBike fleet management needs.
Step 4: Model Validation
After training AI models, it is crucial to validate their performance using test data. Model validation ensures that the models can accurately predict outcomes and provide valuable insights. Employ various evaluation metrics, such as accuracy, precision, and recall, to assess model performance and identify areas for improvement. Fine-tune models as needed to enhance their predictive capabilities and ensure reliable AI-driven decision-making.
Step 5: Model Deployment
Once AI models are trained and validated, they can be deployed within eBike fleet management systems. Integrate AI models with existing software and hardware, ensuring seamless communication and data exchange. Monitor deployed models for performance and accuracy, addressing any issues as they arise. Continuously update and retrain models to maintain their predictive capabilities and adapt to changing fleet conditions.
Real-world examples and best practices can guide the implementation of AI in eBike fleet management. For instance, some fleet managers have successfully integrated AI-powered predictive maintenance systems, reducing downtime and maintenance costs. Others have implemented AI-driven demand forecasting tools, optimizing fleet allocation and improving overall efficiency. By following established best practices and learning from successful implementations, fleet managers can ensure a smooth transition to AI-powered eBike fleet management.
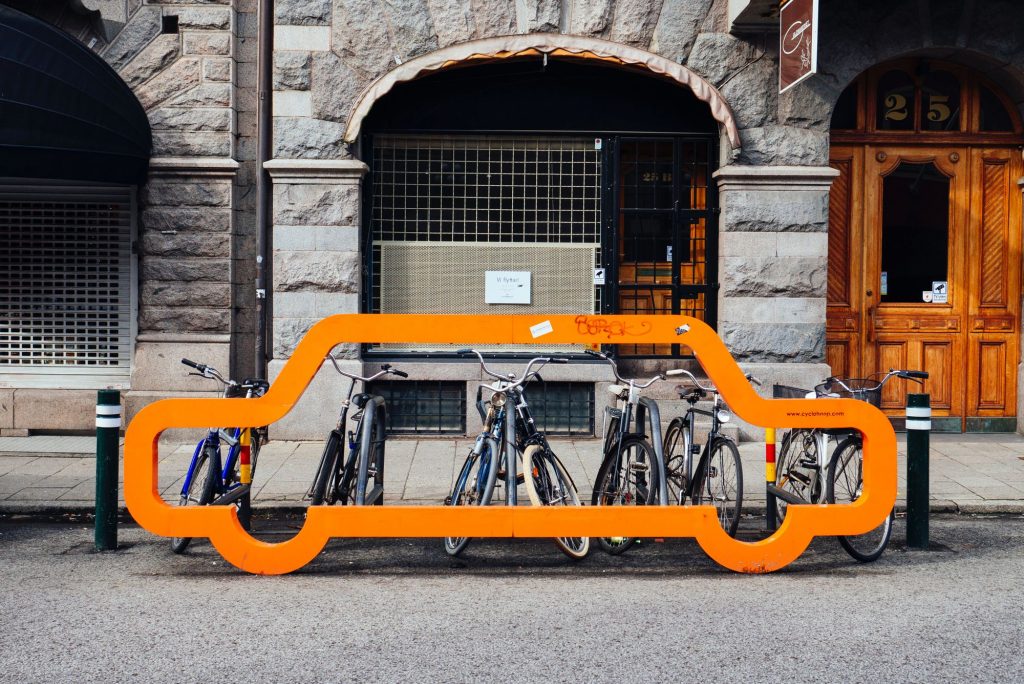
Overcoming Challenges in AI-Driven eBike Fleet Management
Implementing AI in eBike fleet management offers numerous benefits, but it also presents several challenges that fleet managers must address. By understanding these issues and implementing strategies to mitigate them, organizations can ensure a secure, ethical, and successful AI deployment. Here are some potential challenges in AI-driven eBike fleet management and ways to overcome them:
Data Privacy Concerns
AI systems rely on vast amounts of data, raising concerns about user privacy. To address these concerns, ensure that data collection, storage, and processing comply with relevant data protection regulations, such as the General Data Protection Regulation (GDPR) and the California Consumer Privacy Act (CCPA). Implement data anonymization techniques and transparent data usage policies to build trust with users and protect their privacy.
Cybersecurity Threats
AI systems can be vulnerable to cyberattacks, which may compromise sensitive data or disrupt fleet operations. Mitigate these risks by implementing robust cybersecurity measures, such as encryption, firewalls, and intrusion detection systems. Regularly update and patch AI systems to protect against known vulnerabilities and conduct regular security audits to identify and address potential threats.
Regulatory Compliance
AI in eBike fleet management is subject to various regulations, such as those governing transportation, data protection, and safety. Stay informed about relevant regulations and ensure that AI systems comply with all applicable rules. Collaborate with legal experts and regulatory bodies to ensure that AI-driven fleet management practices are compliant and sustainable.
Ethical Considerations
AI systems can inadvertently perpetuate biases or make decisions that have unintended consequences. Address these concerns by incorporating ethical considerations into AI design and deployment. Utilize transparent AI models, conduct regular audits, and establish accountability mechanisms to ensure that AI systems are fair, unbiased, and aligned with organizational values.
By proactively addressing these challenges, fleet managers can ensure a secure, ethical, and successful implementation of AI in eBike fleet management. Employing best practices and collaborating with experts in AI, cybersecurity, and regulation can help organizations navigate the complex landscape of AI-driven fleet management and unlock its full potential.
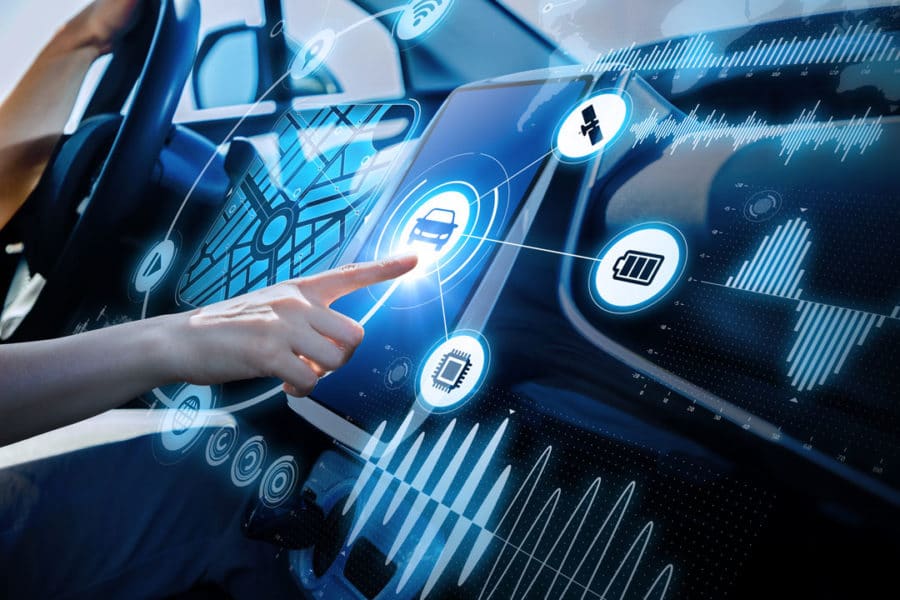
Top AI-Powered eBike Fleet Management Solutions
As AI in eBike fleet management gains traction, several innovative solutions have emerged, offering unique features, benefits, and customer reviews. Here are some of the top AI-driven eBike fleet management solutions that are revolutionizing the industry:
Fleet Complete
Fleet Complete is an all-in-one IoT platform that offers AI-powered solutions for eBike fleets, including real-time tracking, predictive maintenance, and route optimization. With its user-friendly interface and seamless integration with existing infrastructure, Fleet Complete has received positive customer reviews for its ability to improve fleet efficiency and reduce operational costs.
Bosch eBike Systems
Bosch eBike Systems offers an AI-driven connectivity solution called the Bosch eBike Connect app, which enables eBike fleet managers to monitor and analyze fleet performance in real-time. The app provides features such as range prediction, service reminders, and theft protection, making it a popular choice among eBike fleet operators.
COBI.Bike
COBI.Bike is an AI-powered connected biking system that offers eBike fleet management features such as GPS tracking, ride analytics, and theft protection. The system integrates with eBike components, such as lights, motor, and battery, to provide a seamless and efficient fleet management experience. COBI.Bike has received positive customer reviews for its innovative features and ease of use.
Connect Bike
Connect Bike is an AI-powered platform that offers eBike fleet management solutions, including real-time tracking, predictive maintenance, and theft prevention. The platform’s machine learning algorithms analyze fleet data to optimize performance, reduce downtime, and improve overall fleet efficiency. Connect Bike has received positive customer reviews for its advanced analytics and user-friendly interface.
eBikeLabs
eBikeLabs is an AI-driven platform that offers eBike fleet management solutions, including real-time tracking, predictive maintenance, and demand forecasting. The platform’s machine learning algorithms analyze fleet data to optimize performance, reduce downtime, and improve overall fleet efficiency. eBikeLabs has received positive customer reviews for its innovative features and customer support.
These top AI-powered eBike fleet management solutions demonstrate the potential of AI to revolutionize the eBike industry and urban mobility. By leveraging these platforms, eBike fleet operators can improve efficiency, safety, and sustainability, while reducing operational costs and enhancing the user experience.

The Future of AI in eBike Fleet Management
As AI in eBike fleet management continues to evolve, several future trends and developments are expected to revolutionize the industry. These innovations have the potential to further improve efficiency, safety, and sustainability, while transforming urban mobility.
Autonomous eBikes
Autonomous eBikes, powered by AI and advanced sensors, are expected to become a reality in the near future. These self-driving eBikes can navigate urban environments, avoid obstacles, and optimize routes, providing a seamless and efficient transportation experience. Autonomous eBikes can also reduce the need for human intervention, leading to lower operational costs and increased fleet productivity.
Advanced Predictive Analytics
Advanced predictive analytics, powered by AI, can help eBike fleet managers anticipate and address potential issues before they occur. By analyzing historical and real-time data, AI algorithms can predict battery life, maintenance needs, and usage patterns, enabling fleet managers to optimize fleet performance, reduce downtime, and improve overall efficiency.
Integration with Smart City Infrastructure
The integration of AI-powered eBike fleet management systems with smart city infrastructure, such as traffic lights, charging stations, and parking systems, can further enhance the eBike transportation experience. By communicating with smart city systems, eBikes can optimize routes, reduce traffic congestion, and improve overall urban mobility.
Impact on the eBike Industry and Urban Mobility
The future of AI in eBike fleet management has the potential to significantly impact the eBike industry and urban mobility. By improving efficiency, safety, and sustainability, AI-powered eBike fleets can become a preferred mode of transportation in urban areas, reducing the reliance on traditional fossil fuel-powered vehicles. This shift towards eBikes can lead to a cleaner, greener, and more livable urban environment, benefiting both individuals and communities.
In conclusion, the future of AI in eBike fleet management is promising, with several exciting developments on the horizon. By embracing these innovations, eBike fleet operators can improve efficiency, safety, and sustainability, while transforming urban mobility and contributing to a greener future.
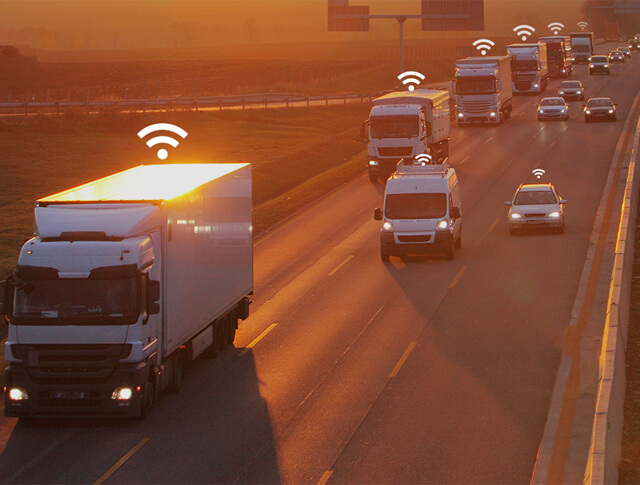
Preparing Your Workforce for AI-Driven eBike Fleet Management
As AI in eBike fleet management becomes increasingly prevalent, it is crucial to prepare your workforce for the transition to these advanced technologies. By upskilling employees and fostering a culture of innovation, you can ensure a smooth and successful integration of AI in eBike fleet management.
Upskilling Employees
Upskilling employees is essential for a successful transition to AI-powered eBike fleet management. By providing training programs and resources, you can help your workforce develop the skills necessary to work alongside AI technologies. This may include training in data analysis, machine learning, and IoT device management. By investing in your employees’ professional development, you can create a more efficient, productive, and adaptable workforce, ready to embrace the future of eBike fleet management.
Fostering a Culture of Innovation
Fostering a culture of innovation is vital for the successful adoption of AI in eBike fleet management. Encourage your employees to explore new ideas, experiment with new technologies, and share their knowledge and experiences. By creating an open and supportive environment, you can empower your workforce to embrace change, adapt to new challenges, and contribute to the ongoing success of your eBike fleet management operations.
Addressing Workforce Concerns
It is essential to address any concerns your workforce may have regarding the transition to AI-powered eBike fleet management. Open communication, transparency, and empathy can help alleviate fears and misconceptions about AI technologies. By actively involving your employees in the decision-making process and demonstrating the benefits of AI in eBike fleet management, you can build trust, increase buy-in, and ensure a smoother transition.
Continuous Learning and Improvement
Continuous learning and improvement are key to maintaining a competitive edge in the rapidly evolving world of AI-driven eBike fleet management. Encourage your employees to stay up-to-date with the latest industry trends, technologies, and best practices. By fostering a culture of continuous learning and improvement, you can ensure that your workforce remains adaptable, innovative, and prepared for the future of eBike fleet management.
In conclusion, preparing your workforce for AI-driven eBike fleet management is crucial for a successful transition to these advanced technologies. By upskilling employees, fostering a culture of innovation, addressing workforce concerns, and promoting continuous learning and improvement, you can ensure a smooth and successful integration of AI in eBike fleet management. This not only benefits your organization but also contributes to a more efficient, sustainable, and innovative eBike industry and urban mobility landscape.