The Role of Artificial Intelligence in eBike Health Monitoring
Artificial Intelligence (AI) has emerged as a game-changer in eBike health monitoring, playing a pivotal role in maintaining eBike performance and longevity. AI-driven monitoring systems offer numerous benefits, such as predictive maintenance, real-time diagnostics, and enhanced safety features. These advancements contribute significantly to the overall rider experience and help reduce maintenance costs.
AI in eBike health monitoring involves the use of machine learning algorithms and data analysis techniques to predict potential issues and optimize eBike performance. By continuously monitoring eBike components, such as batteries, motors, and sensors, AI-powered systems can identify patterns and trends that might indicate future problems. This proactive approach enables riders and technicians to address issues promptly, reducing downtime and repair costs.
Real-time diagnostics is another critical area where AI shines in eBike health monitoring. By analyzing data from various sensors in real-time, AI-driven systems can provide accurate and timely diagnostics, helping riders and technicians quickly identify and resolve issues. This capability not only enhances the rider experience but also ensures optimal eBike performance and safety.
Moreover, AI-based health monitoring systems contribute significantly to eBike safety. By continuously monitoring the overall health of the bike, AI-driven systems can alert riders to potential issues, such as low battery levels, malfunctioning sensors, or potential component failures. These alerts enable riders to take appropriate action, ensuring their safety and preventing costly repairs.
How AI Helps in Predictive Maintenance for eBikes
AI in eBike health monitoring plays a crucial role in predictive maintenance, enabling riders and technicians to anticipate and address potential issues before they escalate. By analyzing historical data and identifying patterns, AI-powered systems can predict potential problems in eBike components, such as batteries, motors, and sensors.
Predictive maintenance in eBikes involves using machine learning algorithms to analyze data from various sensors and systems. For instance, AI can monitor battery health by analyzing data on charge cycles, temperature, and voltage. By identifying trends and patterns in this data, AI-driven systems can predict battery degradation and alert riders to potential issues. Similarly, AI can monitor motor performance, detecting signs of wear and tear or imbalances that might indicate future failures. By predicting these issues, AI-powered systems enable riders and technicians to take proactive maintenance measures, reducing downtime and repair costs.
Real-life examples of AI-powered predictive maintenance solutions in the eBike industry include VanMoof’s Electrified S3 and X3 eBikes. These bikes feature AI-driven systems that monitor battery health, motor performance, and other critical components, predicting potential issues and alerting riders to take action. By employing predictive maintenance, VanMoof has significantly reduced maintenance costs and downtime for its riders.
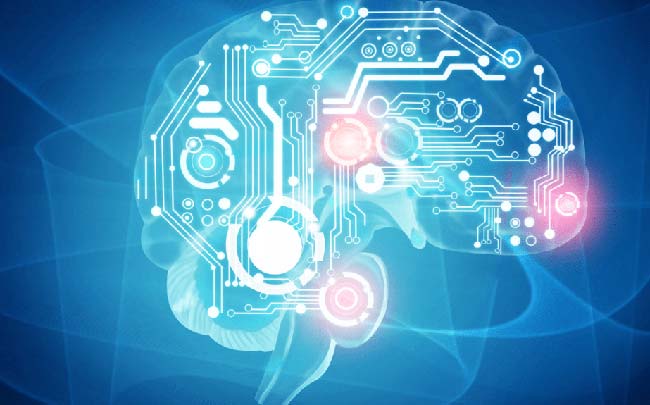
Real-time eBike Diagnostics with AI
AI in eBike health monitoring significantly enhances real-time diagnostics, enabling riders and technicians to identify and address issues promptly. AI-driven diagnostics offer several advantages over traditional methods, including improved accuracy, speed, and convenience.
Traditional diagnostics methods often rely on manual inspections and tests, which can be time-consuming and prone to human error. In contrast, AI-powered systems can analyze data from multiple sensors and systems in real-time, providing accurate and reliable diagnoses. By continuously monitoring eBike components and systems, AI-driven diagnostics can detect issues as soon as they arise, allowing riders and technicians to take immediate action. This swift response can help prevent minor issues from escalating into significant problems, reducing downtime and repair costs.
Moreover, AI-driven diagnostics can provide detailed insights into eBike performance, helping riders and technicians understand the root causes of issues and optimize maintenance strategies. For instance, AI can analyze motor performance data to detect signs of wear and tear, enabling riders and technicians to replace components before they fail. By providing real-time diagnostics, AI-powered systems can help riders and technicians make informed decisions about eBike maintenance, improving overall performance and longevity.
Real-life examples of AI-powered real-time diagnostics in the eBike industry include Cowboy’s eBikes. These bikes feature AI-driven systems that continuously monitor eBike performance, detect issues, and provide real-time diagnostics. By employing real-time diagnostics, Cowboy has improved eBike maintenance and rider experiences, reducing downtime and repair costs.
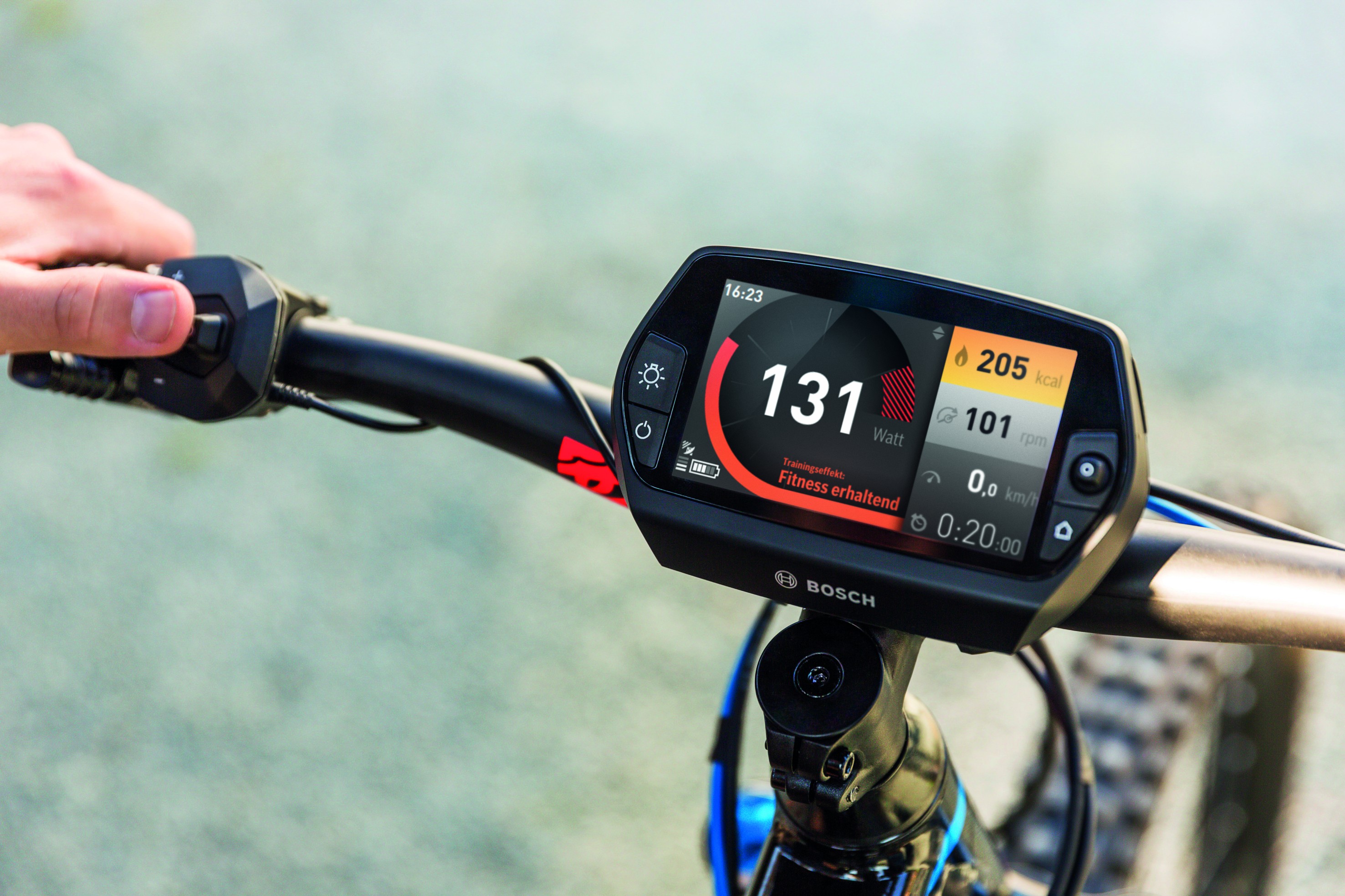
Enhancing eBike Safety with AI-based Health Monitoring
AI plays a crucial role in enhancing eBike safety by continuously monitoring the overall health of the bike. AI-based safety features provide riders and technicians with real-time alerts for low battery levels, malfunctioning sensors, or potential component failures, significantly impacting rider safety.
One of the primary safety concerns in eBikes is the risk of battery failure, which can lead to sudden power loss or even fires. AI-driven health monitoring systems can analyze battery performance data, detect signs of degradation, and predict potential failures before they occur. By providing real-time alerts for low battery levels or imminent battery failures, AI-based systems can help riders avoid dangerous situations and take timely action to replace or repair batteries.
Similarly, AI can monitor eBike sensors and components, detecting malfunctions or potential failures that could compromise rider safety. For instance, AI can analyze motor performance data to detect signs of wear and tear, enabling riders and technicians to replace components before they fail. By continuously monitoring eBike systems, AI-based health monitoring can help prevent accidents and ensure safe riding experiences.
AI-based safety features can also provide riders with valuable insights into eBike performance and maintenance needs. For example, AI can analyze data from eBike sensors to detect issues with braking or steering systems, enabling riders to address these issues promptly and maintain optimal eBike performance. By providing real-time alerts and insights, AI-based safety features can help riders make informed decisions about eBike maintenance and enhance overall safety.
Real-life examples of AI-powered safety features in the eBike industry include VanMoof’s eBikes. These bikes feature AI-driven systems that continuously monitor eBike performance, detect issues, and provide real-time safety alerts. By employing AI-based safety features, VanMoof has improved eBike safety and rider experiences, reducing the risk of accidents and enhancing overall performance.
Popular AI-enabled eBike Health Monitoring Solutions
As AI-driven health monitoring gains traction in the eBike industry, various brands have integrated AI-enabled features into their products. Here are some popular AI-enabled eBike health monitoring solutions:
VanMoof S3 & X3 eBikes
VanMoof’s S3 and X3 eBikes feature AI-driven systems that continuously monitor eBike performance, detect issues, and provide real-time safety alerts. The bikes also include anti-theft features, such as location tracking and remote locking, which leverage AI technology to enhance security.
Cowboy 4 eBike
Cowboy’s fourth-generation eBike features AI-powered diagnostics and predictive maintenance capabilities. The bike’s integrated sensors collect data on various components, including the battery, motor, and brakes, enabling the AI system to detect potential issues and alert riders before they become serious problems. Cowboy’s mobile app also provides real-time insights into eBike performance and maintenance needs.
THOK TK01
THOK’s TK01 eBike features an AI-powered torque sensor that optimizes pedal assistance based on rider input. The sensor analyzes data from the bike’s motor and battery, delivering a smooth and responsive riding experience. Additionally, the TK01’s integrated display provides real-time data on battery levels, speed, and distance, enabling riders to monitor eBike performance and maintain optimal conditions.
Connect Bike
Connect Bike offers an AI-enabled eBike health monitoring solution that integrates with various eBike brands. The system includes sensors that attach to eBike components, collecting data on battery levels, motor performance, and other critical metrics. The AI system analyzes this data, providing real-time alerts and insights into eBike performance and maintenance needs. Connect Bike’s mobile app also enables riders to track their rides, monitor eBike health, and receive safety alerts.
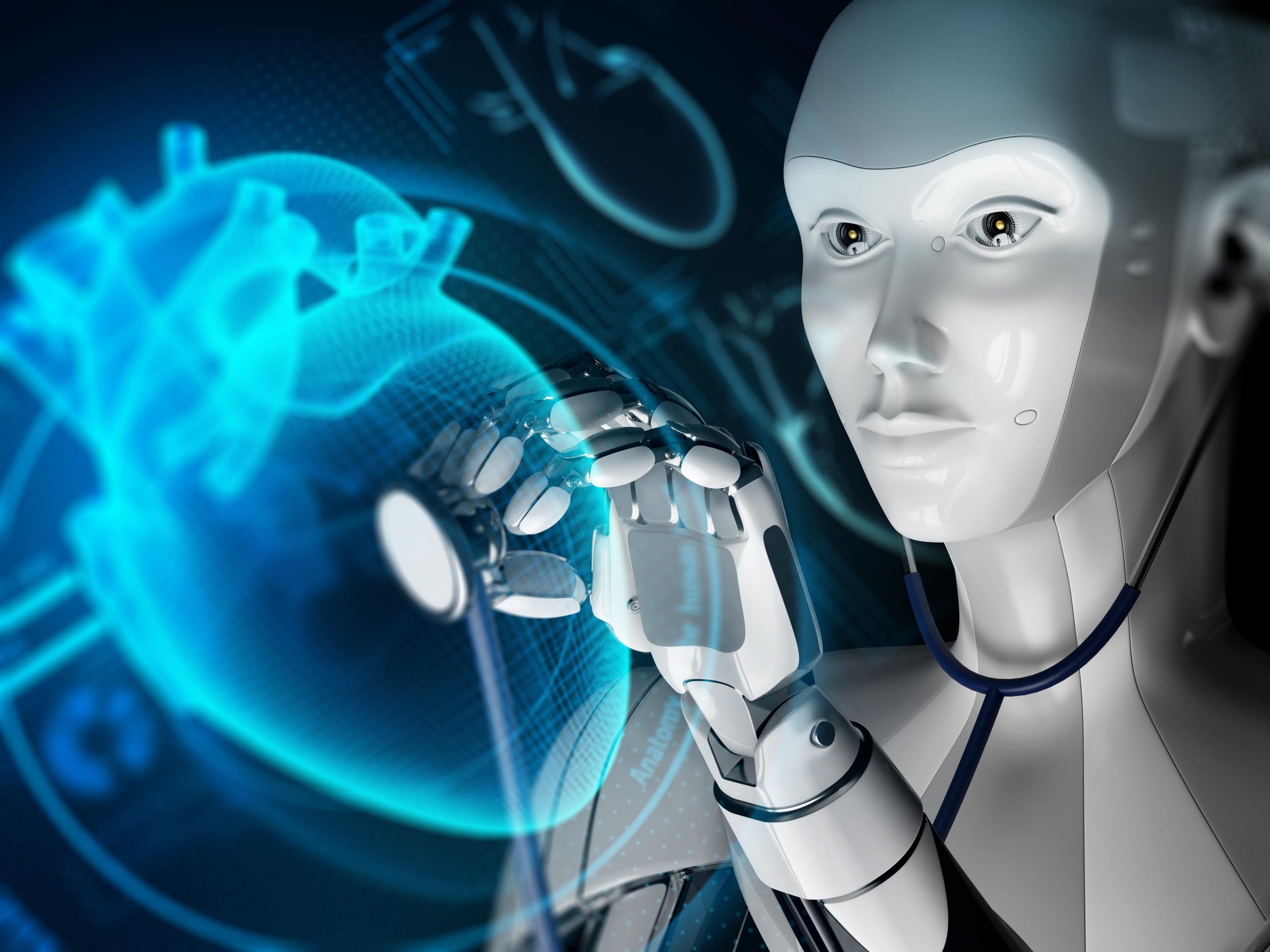
Future Perspectives: AI in eBike Health Monitoring
As AI technology continues to advance, the potential for AI in eBike health monitoring is vast and promising. Here are some key areas where AI could revolutionize eBike maintenance and rider experiences:
Improved Machine Learning Algorithms
Machine learning algorithms are at the core of AI-driven eBike health monitoring. As these algorithms become more sophisticated, they will be better equipped to analyze complex data patterns and predict potential issues with greater accuracy. Improved machine learning algorithms could also enable AI systems to learn from the collective experiences of multiple eBikes, further enhancing their predictive capabilities.
Advanced Sensor Technology
Advancements in sensor technology will enable AI systems to collect more detailed and accurate data on eBike performance. This data can then be used to develop more precise predictive maintenance models and real-time diagnostic tools. Additionally, the integration of advanced sensors, such as those that measure road conditions or weather patterns, could enable AI systems to provide riders with personalized safety recommendations and optimized riding experiences.
Enhanced Data Analysis Capabilities
As AI systems become more sophisticated, they will be better equipped to analyze large volumes of data in real-time. This enhanced data analysis capability could enable AI systems to provide riders and technicians with more detailed insights into eBike performance and maintenance needs. Additionally, the integration of natural language processing (NLP) technology could enable AI systems to communicate these insights in a more user-friendly and accessible manner.
Integration with Smart Cities and IoT Devices
As eBikes become increasingly integrated with smart cities and IoT devices, AI systems could be used to optimize eBike performance based on real-time data from these systems. For example, AI systems could use data from traffic sensors to optimize eBike routes, reducing travel time and energy consumption. Additionally, the integration of AI systems with other IoT devices, such as smart homes or wearable technology, could enable riders to monitor eBike performance and maintenance needs from a single platform.
Personalized Riding Experiences
AI systems could be used to create personalized riding experiences based on rider preferences and habits. For example, AI systems could use data on rider behavior to optimize pedal assistance, providing a smoother and more enjoyable riding experience. Additionally, AI systems could provide riders with personalized safety recommendations based on real-time data, such as weather conditions or road conditions.
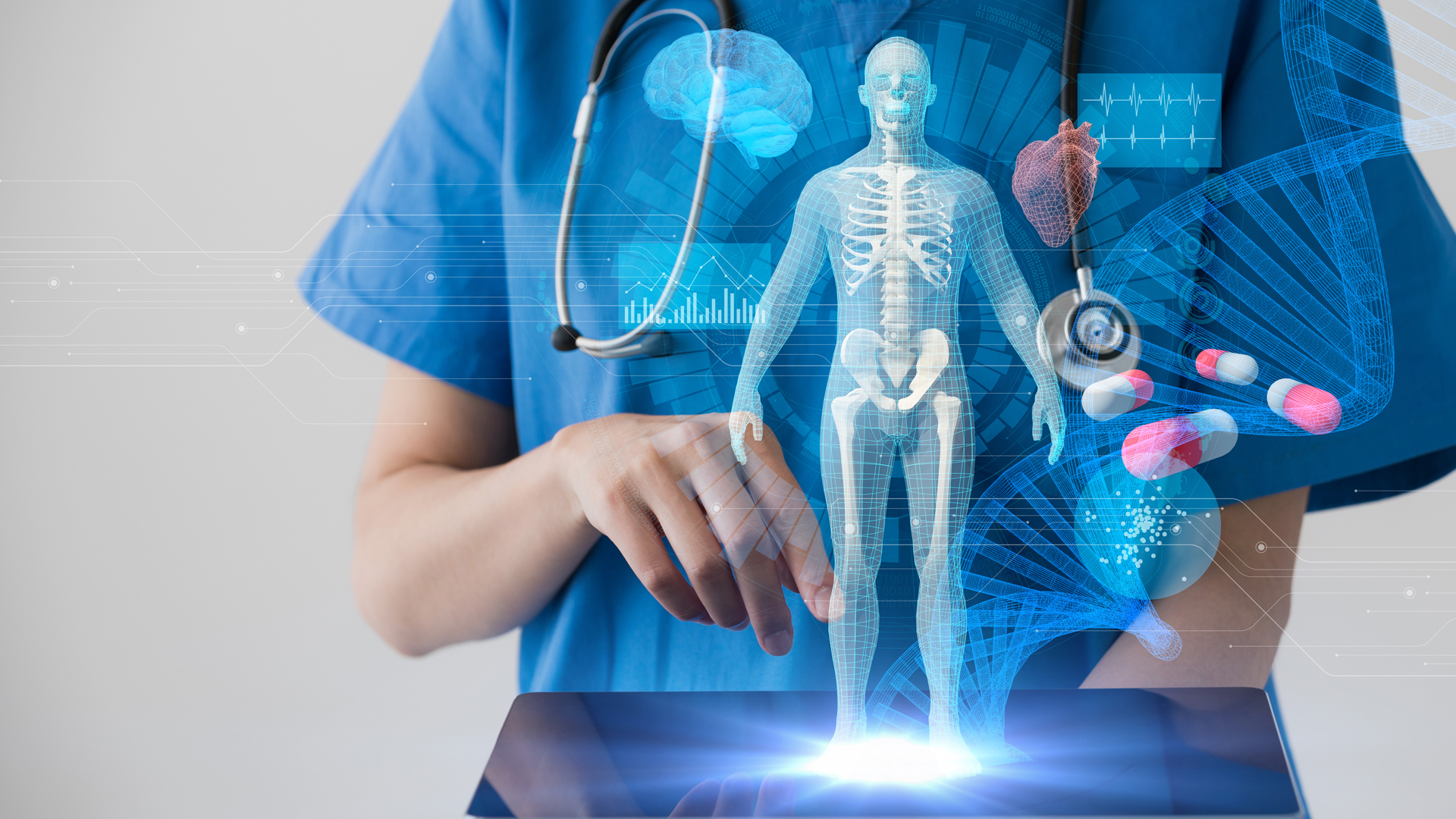
Challenges and Limitations of AI in eBike Health Monitoring
Despite the numerous benefits of AI in eBike health monitoring, there are challenges and limitations that need to be addressed to ensure the successful deployment and adoption of these innovative solutions. Here are some of the key issues and potential solutions:
Data Privacy Concerns
One of the major concerns with AI in eBike health monitoring is the collection and use of sensitive data. Riders may be hesitant to share their riding patterns, location data, and other personal information with third-party service providers. To address this issue, eBike manufacturers and service providers must ensure that they comply with data protection regulations and provide clear and transparent privacy policies. They should also consider implementing data anonymization techniques to protect user privacy while still enabling AI-driven insights.
System Costs
The integration of AI in eBike health monitoring systems can be expensive, especially for high-end features such as predictive maintenance and real-time diagnostics. This can be a barrier to entry for some eBike manufacturers and riders. To overcome this challenge, manufacturers can consider offering AI-enabled monitoring solutions as optional features or as part of premium packages. They can also explore partnerships with third-party service providers to offer affordable AI-driven monitoring services to riders.
User Acceptance
Another challenge is user acceptance of AI-driven monitoring solutions. Some riders may be skeptical of the benefits of AI or may prefer traditional monitoring methods. To address this issue, eBike manufacturers and service providers must focus on user education and training. They should provide clear and concise instructions on how to use AI-enabled monitoring solutions and highlight the benefits of these features. They can also offer user support and feedback mechanisms to address any concerns or issues that may arise.
Continuous Innovation and Development
Finally, the field of AI in eBike health monitoring is constantly evolving, with new technologies and approaches emerging regularly. To stay competitive, eBike manufacturers and service providers must invest in continuous research and development. They should explore new machine learning algorithms, sensor technologies, and data analysis techniques to improve the accuracy, speed, and convenience of AI-driven monitoring solutions. By doing so, they can ensure that they remain at the forefront of the eBike industry and provide the best possible experiences for their customers.
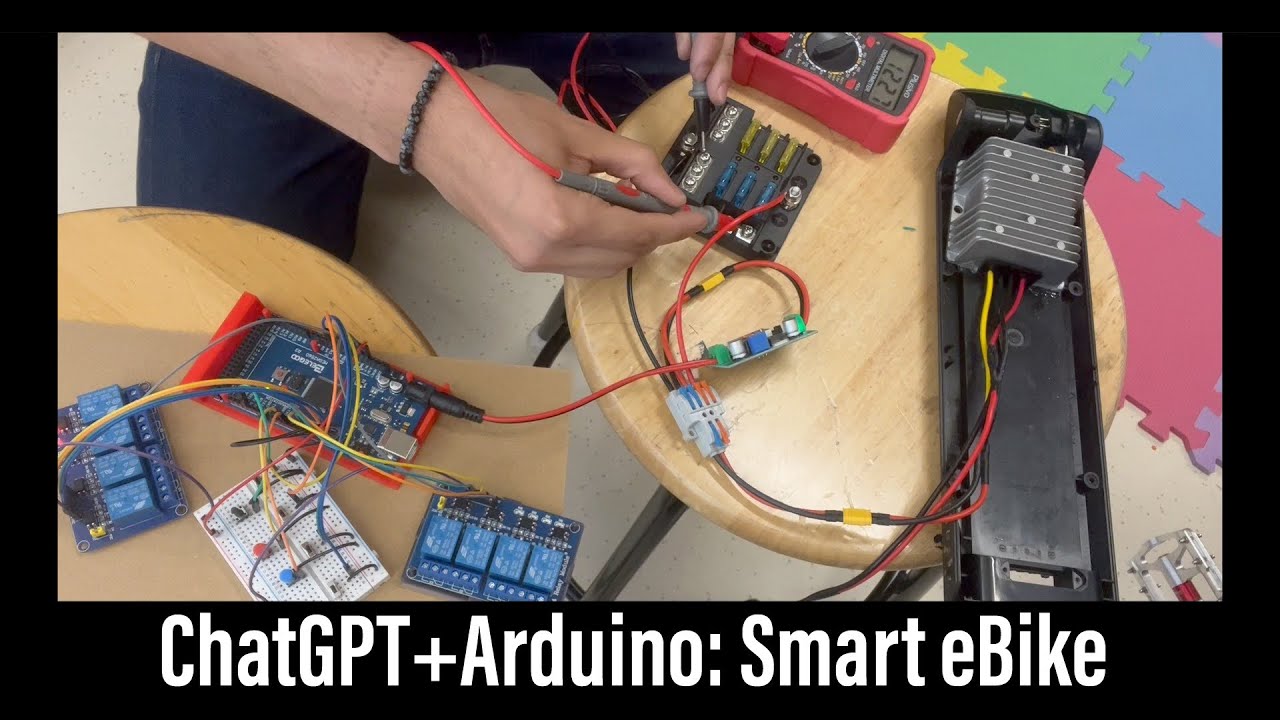
Implementing AI in eBike Health Monitoring: Best Practices
As AI continues to play an increasingly significant role in eBike health monitoring, it is essential to follow best practices to ensure the successful deployment and adoption of these innovative solutions. Here are some key factors to consider when implementing AI in eBike health monitoring:
Data Management
Effective data management is critical for the success of AI-driven monitoring systems. Manufacturers and service providers must ensure that they collect, store, and analyze high-quality data from eBike sensors and components. They should also consider data privacy and security issues and implement appropriate measures to protect user data. By doing so, they can ensure that their AI-enabled monitoring solutions provide accurate and reliable insights into eBike health and performance.
System Integration
Integrating AI-driven monitoring systems with existing eBike hardware and software can be challenging. Manufacturers and service providers must ensure that their AI-enabled solutions are compatible with different eBike models and brands. They should also consider the user experience and ensure that their monitoring solutions are easy to use and integrate seamlessly with other eBike features. By doing so, they can provide a seamless and enjoyable riding experience for eBike users.
User Training
User training is essential for the successful adoption of AI-enabled monitoring solutions. Manufacturers and service providers must provide clear and concise instructions on how to use their monitoring solutions and highlight the benefits of these features. They should also offer user support and feedback mechanisms to address any concerns or issues that may arise. By doing so, they can ensure that eBike users are comfortable and confident when using AI-driven monitoring solutions.
Continuous Innovation and Development
Finally, the field of AI in eBike health monitoring is constantly evolving, with new technologies and approaches emerging regularly. Manufacturers and service providers must invest in continuous research and development to stay competitive and provide the best possible experiences for their customers. They should explore new machine learning algorithms, sensor technologies, and data analysis techniques to improve the accuracy, speed, and convenience of AI-driven monitoring solutions. By doing so, they can ensure that they remain at the forefront of the eBike industry and provide the best possible experiences for their customers.
In conclusion, implementing AI in eBike health monitoring requires careful consideration of data management, system integration, user training, and continuous innovation and development. By following these best practices, eBike manufacturers and service providers can ensure the successful deployment and adoption of AI-driven monitoring solutions, providing a safer, more enjoyable, and more efficient riding experience for eBike users.
