Understanding Artificial Intelligence for eBike Component Lifespan
Artificial Intelligence (AI) is a rapidly evolving technology that has the potential to revolutionize various industries, including eBike maintenance. AI technology can be used to improve eBike component lifespan by analyzing sensor data and predicting potential failures before they occur. This proactive approach to maintenance can lead to reduced downtime, lower maintenance costs, and increased safety for eBike users.
AI technology can be applied to eBike maintenance through the use of machine learning algorithms, predictive analytics, and sensor data. Machine learning algorithms can analyze large amounts of data from sensors installed on eBikes to identify patterns and trends that may indicate potential issues with eBike components. Predictive analytics can then be used to forecast when these issues are likely to occur, allowing maintenance to be scheduled proactively.
Sensor data is a critical component of AI-powered eBike maintenance. Sensors can be installed on various eBike components, such as the battery, motor, and brakes, to monitor their performance and detect any anomalies. This data can then be analyzed using machine learning algorithms to identify potential issues and predict component failures before they occur. By leveraging AI technology in this way, eBike manufacturers and maintenance providers can improve eBike component lifespan and provide a safer, more reliable riding experience for eBike users.
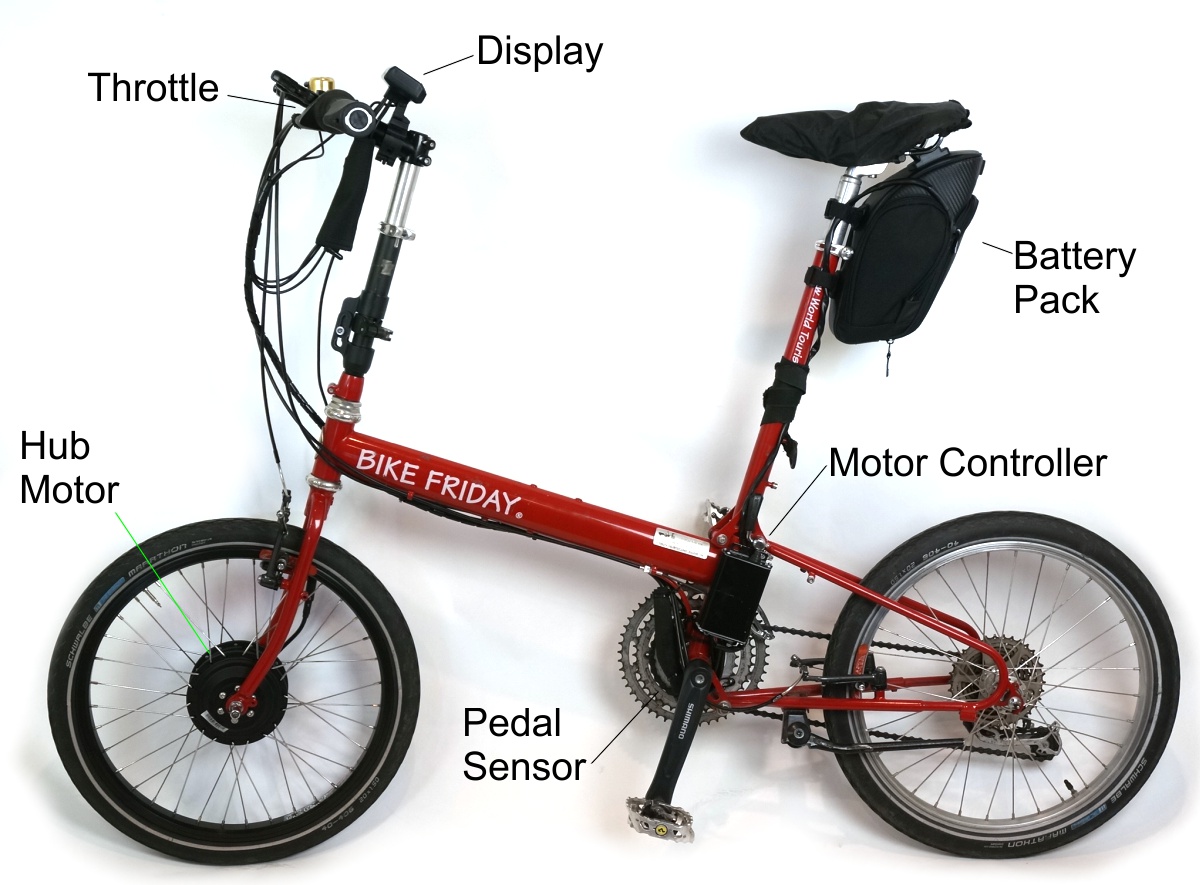
The Role of AI in Predictive Maintenance for eBike Component Lifespan
Artificial intelligence (AI) has the potential to revolutionize eBike maintenance by enabling predictive maintenance of eBike components. Predictive maintenance involves using machine learning algorithms to analyze sensor data and predict potential failures before they occur, allowing for proactive maintenance and reducing downtime, lowering maintenance costs, and increasing safety.
Traditional reactive maintenance involves waiting for components to fail before repairing or replacing them, which can lead to unexpected downtime and higher maintenance costs. Predictive maintenance, on the other hand, enables maintenance to be scheduled proactively, reducing the likelihood of unexpected failures and minimizing downtime. This approach can also lead to lower maintenance costs, as components can be replaced before they fail completely, reducing the need for emergency repairs.
Machine learning algorithms are a key component of predictive maintenance for eBike component lifespan. These algorithms can analyze large amounts of sensor data to identify patterns and trends that may indicate potential issues with eBike components. For example, machine learning algorithms can detect anomalies in battery health or motor performance, allowing maintenance to be scheduled before a failure occurs. By using machine learning algorithms in this way, eBike manufacturers and maintenance providers can improve eBike component lifespan and provide a safer, more reliable riding experience for eBike users.
Sensor data is critical for predictive maintenance of eBike components. Sensors can be installed on various eBike components, such as the battery, motor, and brakes, to monitor their performance and detect any anomalies. This data can then be analyzed using machine learning algorithms to identify potential issues and predict component failures before they occur. By leveraging AI technology in this way, eBike manufacturers and maintenance providers can improve eBike component lifespan and provide a safer, more reliable riding experience for eBike users.
Real-World Applications of AI in eBike Maintenance
Artificial intelligence (AI) technology has already been implemented in various eBike maintenance applications, demonstrating its potential to improve eBike component lifespan and overall performance. By leveraging AI-powered sensors and machine learning algorithms, eBike manufacturers and maintenance providers can monitor and maintain eBike components more effectively, reducing downtime, lowering maintenance costs, and increasing safety.
One example of AI in eBike maintenance is the use of AI-powered sensors to monitor battery health. Battery performance is critical for eBike range and reliability, and AI sensors can detect anomalies in battery health before they become significant issues. For instance, Bosch eBike Systems has implemented AI technology in their batteries to monitor cell health and predict potential failures before they occur. This proactive maintenance approach can significantly improve eBike component lifespan and reduce maintenance costs.
Another application of AI in eBike maintenance is the monitoring of motor performance. eBike motors are complex systems that require regular maintenance to ensure optimal performance. AI sensors can monitor motor performance in real-time, detecting any anomalies that may indicate potential issues. For example, the Specialized Turbo Levo eBike uses AI sensors to monitor motor temperature and adjust power output accordingly, reducing wear and tear on the motor and improving overall performance.
Other critical eBike components, such as brakes and suspension systems, can also benefit from AI-powered sensors and machine learning algorithms. By continuously monitoring these components, eBike manufacturers and maintenance providers can detect potential issues before they become significant problems, reducing downtime and lowering maintenance costs. Additionally, AI technology can provide real-time feedback to eBike users, helping them optimize their riding style and extend the lifespan of their eBike components.
In summary, AI technology has numerous real-world applications in eBike maintenance, from monitoring battery health to optimizing motor performance. By leveraging AI-powered sensors and machine learning algorithms, eBike manufacturers and maintenance providers can improve eBike component lifespan, reduce downtime, lower maintenance costs, and increase safety. As AI technology continues to evolve, we can expect to see even more innovative applications in eBike maintenance and design, providing significant benefits for eBike users and the industry as a whole.
How to Implement Artificial Intelligence in eBike Maintenance
Implementing artificial intelligence (AI) in eBike maintenance can provide numerous benefits, including improved component lifespan, reduced downtime, and lower maintenance costs. However, successfully implementing AI technology requires careful planning and execution. Here are the steps involved in implementing AI in eBike maintenance:
1. Selecting appropriate sensors: The first step in implementing AI in eBike maintenance is selecting appropriate sensors to collect data on eBike components. These sensors should be able to collect accurate and reliable data on critical components such as batteries, motors, brakes, and suspension systems. When selecting sensors, consider factors such as cost, accuracy, and compatibility with existing eBike systems.
2. Data analysis tools: Once sensors have been selected, the next step is to choose data analysis tools to process and analyze the collected data. These tools should be able to handle large amounts of data and provide real-time insights into eBike component health. Consider factors such as ease of use, compatibility with sensors, and cost when selecting data analysis tools.
3. Machine learning algorithms: Machine learning algorithms are critical to the success of AI in eBike maintenance. These algorithms can analyze sensor data and predict potential failures before they occur, allowing for proactive maintenance and reduced downtime. When selecting machine learning algorithms, consider factors such as accuracy, reliability, and compatibility with data analysis tools.
4. Integration with existing maintenance processes: Once sensors, data analysis tools, and machine learning algorithms have been selected, the next step is to integrate AI technology into existing eBike maintenance processes. This may involve training maintenance staff on the use of new tools and technologies, as well as updating maintenance procedures to incorporate AI insights. Consider factors such as staff training, process changes, and cost when integrating AI technology into existing maintenance processes.
5. Ongoing monitoring and evaluation: Finally, it is essential to continuously monitor and evaluate the performance of AI technology in eBike maintenance. This may involve tracking component lifespan, downtime, and maintenance costs, as well as soliciting feedback from maintenance staff. Regular monitoring and evaluation can help identify areas for improvement and ensure the ongoing success of AI in eBike maintenance.
In summary, implementing AI in eBike maintenance requires careful planning and execution. By selecting appropriate sensors, data analysis tools, and machine learning algorithms, and integrating AI technology into existing maintenance processes, eBike manufacturers and maintenance providers can improve component lifespan, reduce downtime, and lower maintenance costs. Ongoing monitoring and evaluation are also critical to ensure the ongoing success of AI in eBike maintenance.
Challenges and Limitations of AI in eBike Maintenance
While artificial intelligence (AI) has the potential to significantly improve eBike component lifespan, there are also challenges and limitations to consider. Here are some of the key challenges and limitations of using AI in eBike maintenance:
1. Accurate and reliable sensor data: AI technology relies on accurate and reliable sensor data to make predictions and provide insights. However, sensors can be prone to errors and inaccuracies, which can lead to false positives or negatives. To address this challenge, it is important to use multiple sensors and data sources to ensure the accuracy and reliability of the data. Additionally, ongoing calibration and maintenance of sensors can help ensure their accuracy over time.
2. Potential for false positives or negatives: AI algorithms are not infallible and can sometimes produce false positives or negatives. A false positive may result in unnecessary maintenance or component replacement, while a false negative could lead to component failure and safety issues. To mitigate this risk, it is important to use machine learning algorithms that are proven to be accurate and reliable, and to continuously monitor and evaluate the performance of the AI system.
3. Cost and complexity of implementing AI technology: Implementing AI technology in eBike maintenance can be costly and complex, requiring significant investment in sensors, data analysis tools, and machine learning algorithms. Additionally, integrating AI technology into existing maintenance processes can be challenging, requiring changes to maintenance procedures and staff training. To address this challenge, it is important to carefully evaluate the potential benefits and costs of AI technology, and to develop a clear implementation plan that includes staff training and process changes.
4. Ethical considerations: There are also ethical considerations to consider when using AI in eBike maintenance. For example, there is the potential for bias in machine learning algorithms, which could lead to unfair treatment of certain eBike components or users. Additionally, the use of AI technology could impact jobs and employment, as some maintenance tasks may be automated. To address these ethical concerns, it is important to ensure transparency and accountability in the use of AI technology, and to develop industry standards and regulations that promote the responsible use of AI.
In summary, while AI has the potential to significantly improve eBike component lifespan, there are also challenges and limitations to consider. These include the need for accurate and reliable sensor data, the potential for false positives or negatives, the cost and complexity of implementing AI technology, and ethical considerations. By addressing these challenges and limitations, eBike manufacturers and maintenance providers can ensure the safe and effective use of AI technology in eBike maintenance.

The Future of AI in eBike Maintenance
Artificial intelligence (AI) has the potential to significantly transform eBike maintenance, offering numerous benefits for eBike users and manufacturers alike. As AI technology continues to advance, we can expect to see increased automation, improved accuracy and reliability, and the integration of AI technology into other aspects of eBike design and functionality.
One of the key benefits of AI in eBike maintenance is the potential for increased automation. With AI-powered sensors and data analysis tools, eBike maintenance can be automated to a large extent, reducing the need for manual intervention and human error. This can lead to reduced downtime, lower maintenance costs, and increased safety for eBike users. Additionally, AI-powered maintenance systems can provide real-time alerts and notifications, allowing eBike users to take proactive action to address potential issues before they become major problems.
Another benefit of AI in eBike maintenance is improved accuracy and reliability. AI algorithms can analyze large amounts of data from multiple sensors, providing more accurate and reliable predictions about potential failures and maintenance needs. This can help eBike manufacturers and maintenance providers to identify and address issues before they become major problems, reducing the risk of component failure and improving overall eBike performance.
As AI technology continues to advance, we can also expect to see the integration of AI technology into other aspects of eBike design and functionality. For example, AI-powered navigation systems can help eBike users to find the most efficient and safe routes, while AI-powered safety features can help to prevent accidents and protect eBike users. Additionally, AI-powered performance tracking systems can help eBike users to monitor their performance and make data-driven decisions about their training and riding habits.
The potential benefits of AI for eBike component lifespan are significant, and eBike manufacturers and users are increasingly recognizing the value of AI technology in eBike maintenance. However, there are also challenges and limitations to consider, such as the need for accurate and reliable sensor data, the potential for false positives or negatives, and the cost and complexity of implementing AI technology. To address these challenges, it is important to continue ongoing research and development in the field of AI for eBike component lifespan, and to develop industry standards and regulations that promote the responsible use of AI technology.
In conclusion, the future of AI in eBike maintenance is bright, with the potential for increased automation, improved accuracy and reliability, and the integration of AI technology into other aspects of eBike design and functionality. By continuing to invest in research and development, and by addressing the challenges and limitations of AI technology, we can unlock the full potential of AI for eBike component lifespan, transforming eBike maintenance and performance for the better.
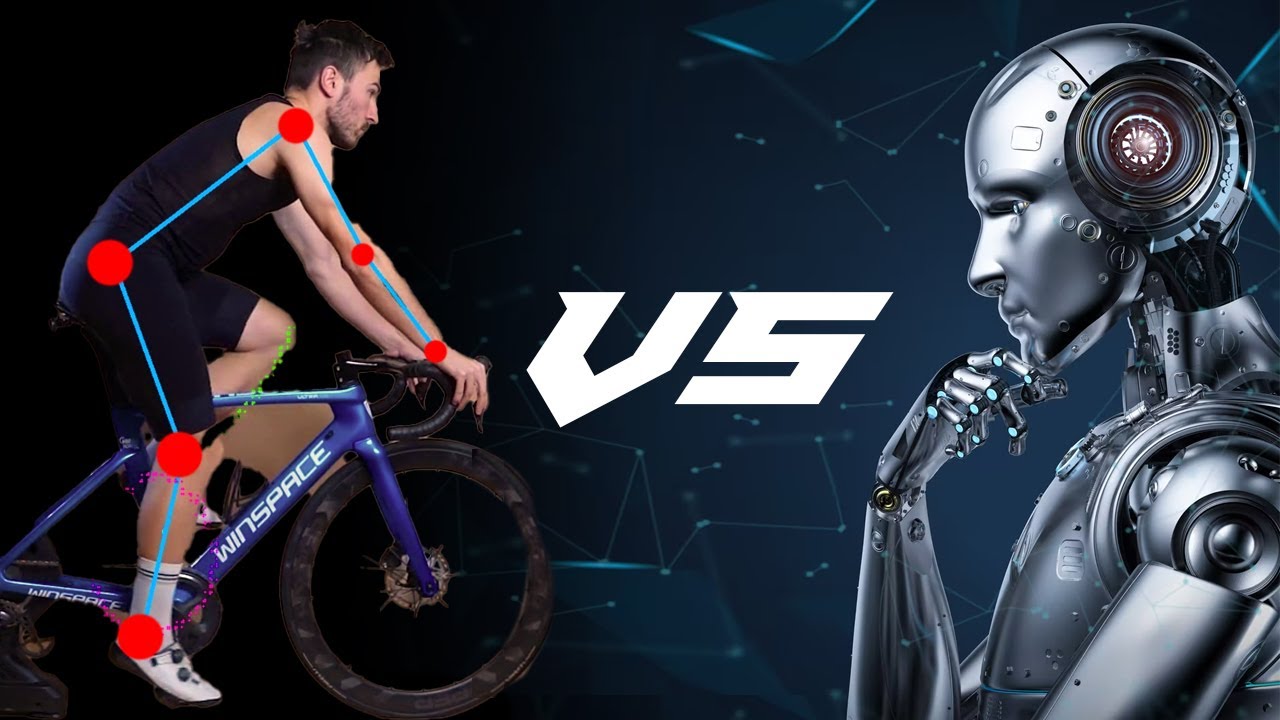
Ethical Considerations of AI in eBike Maintenance
As artificial intelligence (AI) technology continues to advance and become more prevalent in eBike maintenance, it is important to consider the ethical implications of its use. Here are some of the key ethical considerations of AI in eBike component lifespan:
- Bias in machine learning algorithms: Machine learning algorithms are trained on data, and if the data is biased, the algorithms can also become biased. This can lead to unfair or discriminatory outcomes in eBike maintenance, such as prioritizing maintenance for certain users or components over others. To address this challenge, it is important to ensure that the data used to train machine learning algorithms is representative and unbiased, and to regularly evaluate and update the algorithms to minimize bias.
- Impact on jobs and employment: The use of AI in eBike maintenance has the potential to automate certain tasks and reduce the need for human intervention. While this can lead to cost savings and increased efficiency, it can also impact jobs and employment in the eBike industry. To mitigate this impact, it is important to ensure that the transition to AI-powered maintenance is done in a way that supports workers and provides opportunities for re-skilling and up-skilling. Additionally, eBike manufacturers and maintenance providers should consider the potential social and economic impacts of AI technology, and work to ensure that its benefits are shared equitably.
- Transparency and accountability: As AI technology becomes more complex, it can become difficult to understand how decisions are being made and why. This lack of transparency can lead to mistrust and skepticism, and can make it difficult to hold the technology accountable for its actions. To address this challenge, it is important to ensure that AI technology is transparent and explainable, and that there are clear mechanisms for accountability and redress. This can include providing clear explanations of how AI algorithms work, and establishing clear guidelines and regulations for their use.
By addressing these ethical considerations, eBike manufacturers and maintenance providers can help to ensure that the use of AI technology is responsible, equitable, and beneficial for all stakeholders. This can help to build trust and confidence in AI technology, and can pave the way for its successful integration into eBike maintenance and performance.
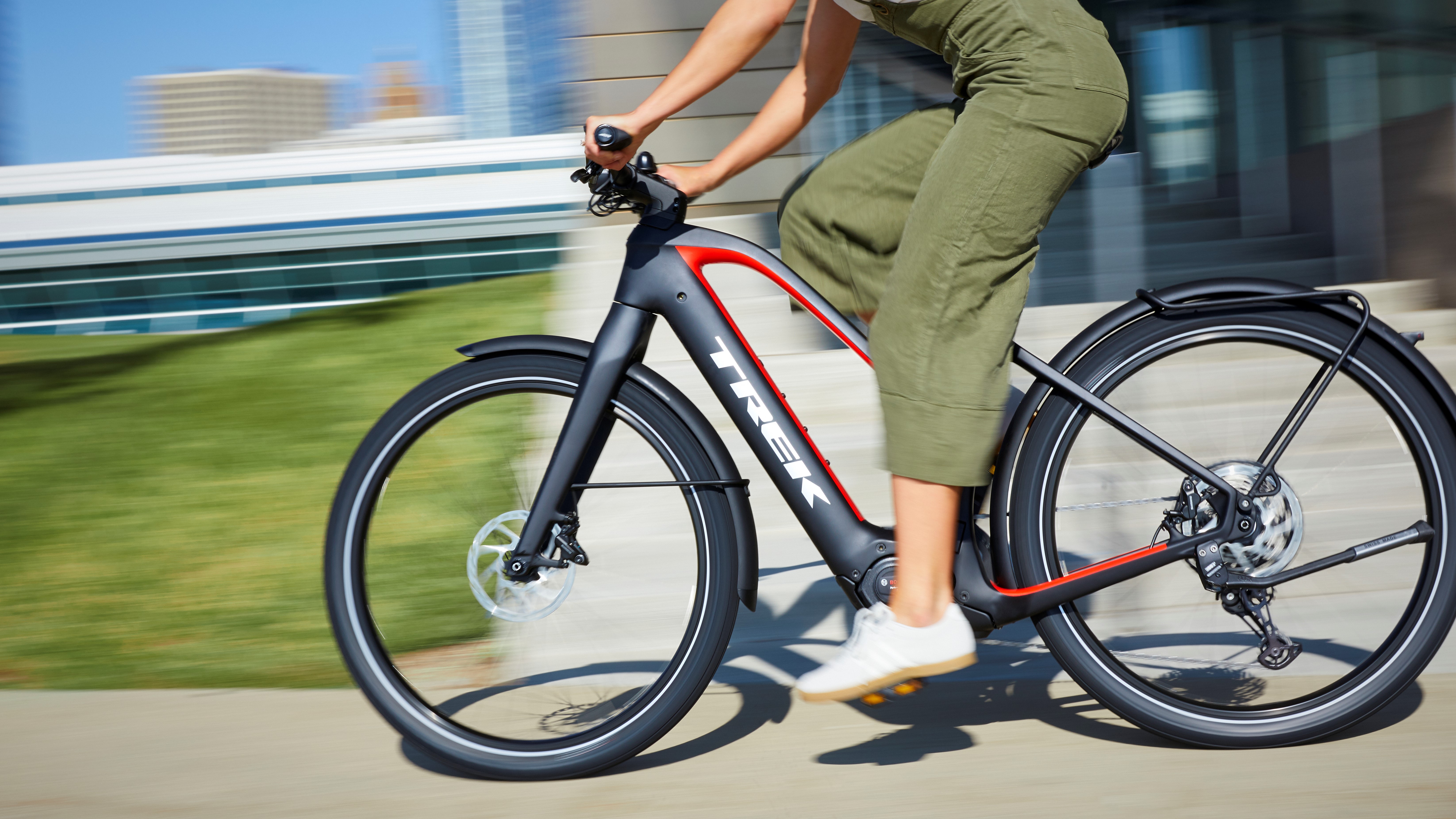
Conclusion: The Benefits of AI for eBike Component Lifespan
Artificial intelligence (AI) technology has the potential to revolutionize eBike maintenance and performance, particularly in the area of eBike component lifespan. By leveraging AI, eBike manufacturers and users can benefit from improved predictive maintenance, reduced downtime, lower maintenance costs, and increased safety.
Through the use of machine learning algorithms, predictive analytics, and sensor data, AI can help to monitor and maintain eBike components, detecting potential failures before they occur and preventing costly and time-consuming repairs. Real-world applications of AI in eBike maintenance include the use of AI-powered sensors to monitor battery health, motor performance, and other critical components.
Implementing AI in eBike maintenance involves selecting appropriate sensors, data analysis tools, and machine learning algorithms, and integrating them into existing maintenance processes. While there are challenges and limitations to using AI in eBike maintenance, such as the need for accurate and reliable sensor data and the cost and complexity of implementation, potential solutions include the use of multiple sensors and data sources and ongoing research and development.
The future of AI in eBike maintenance is promising, with the potential for increased automation, improved accuracy and reliability, and the integration of AI technology into other aspects of eBike design and functionality. The benefits of AI for eBike users include increased safety, reduced maintenance costs, and improved overall performance.
However, it is important to address the ethical considerations of using AI in eBike maintenance, such as the potential for bias in machine learning algorithms, the impact on jobs and employment, and the need for transparency and accountability. Industry standards and regulations can play a crucial role in ensuring the responsible use of AI technology.
In conclusion, the use of AI for eBike component lifespan has the potential to transform eBike maintenance and performance, providing significant benefits for both manufacturers and users. By exploring the potential of AI and addressing the challenges and ethical considerations, we can unlock the full potential of this exciting technology for the eBike industry.
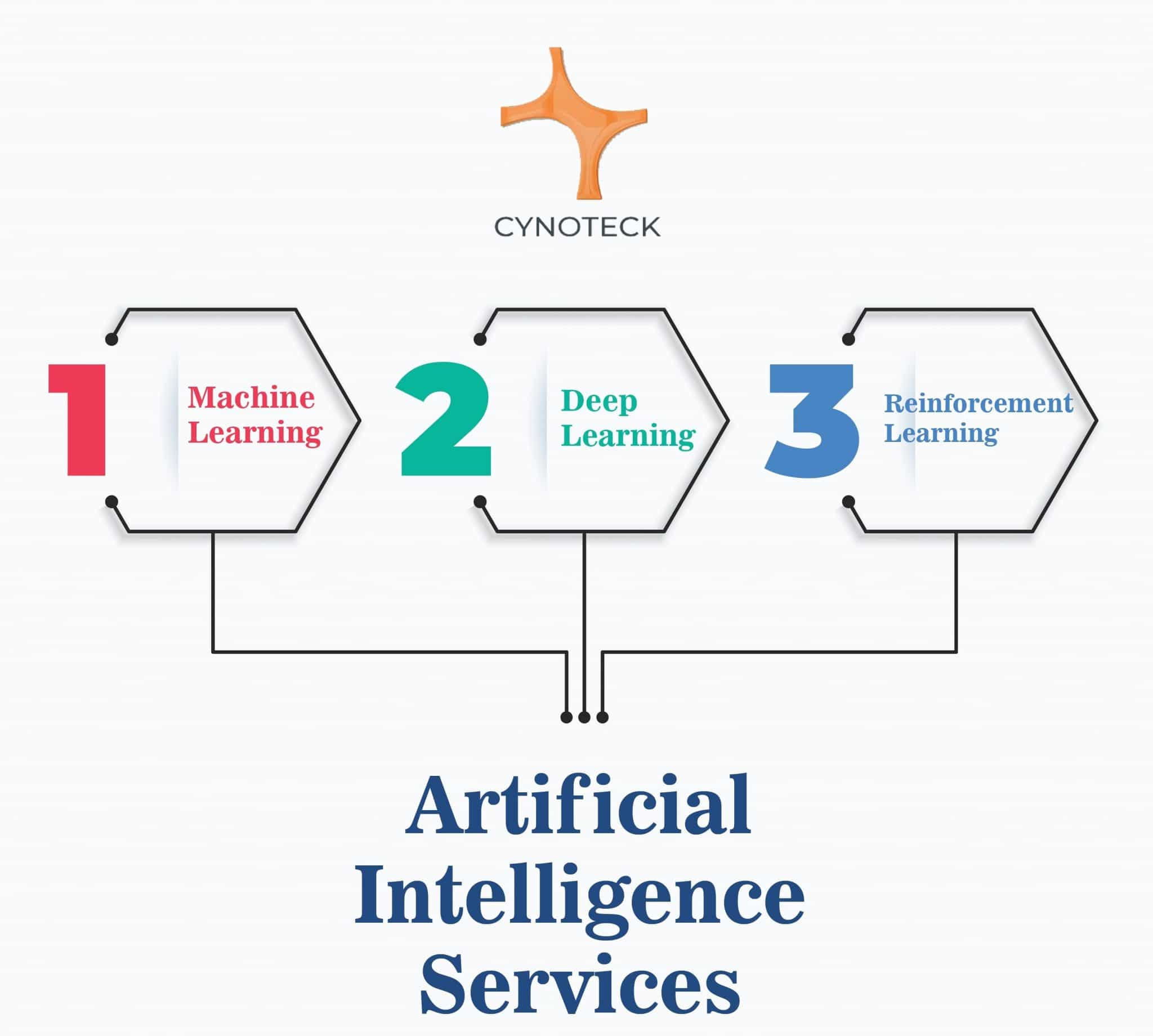